by Andres Müller & Stefan Rädiker
Generative AI offers new possibilities and challenges for qualitative researchers, as recently discussed in a symposium at the MAXQDA user conference, MQIC 2024, in Berlin. With the increasing popularity of genera-tive AI, large language models (LLMs) have now been integrated into well-established research software such as MAXQDA. Since the first release of MAXQDA’s add-on “AI Assist” in April 2023, feature after feature has been added, considerably reducing the barriers for researchers to leverage the capabilities of generative AI in the form of large language models in their data analysis. AI Assist now offers researchers a range of supporting functions, including automatic summaries of various data sections, suggestions for code systems, and explanations of unfamiliar terms. With the release of MAXQDA 24.3, the software now incorporates the popular interaction logic of ChatGPT. This addition transforms MAXQDA from a tool primarily centered around coding data to one that also enables researchers to “chat” with AI about specific documents or selections of coded segments.
While automatic summaries and code suggestions are straightforward to use and seamlessly integrate into established methods such as qualitative content analysis, thematic analysis, grounded theory, or discourse analysis, the chat features open up a new universe of analytical possibilities. Although these features can be integrated into existing methodological approaches (as, for example, described by Kuckartz & Rädiker, in press), they would also benefit from new guidelines and best practices for effective use in analytical contexts.
This blog post aims to address exactly this gap in guidance by presenting ten prompts related to ten use cases: five for case-based analysis and five for theme-based analysis. While our examples focus on interviews, the prompts can be easily adapted for other data types.
Chatting or Prompting?
What does it mean to “chat” with data? The Oxford Dictionary offers two definitions for this seemingly innocent term. The first, “to talk in a friendly, informal way,” doesn’t accurately describe the interactive process that researchers apply in a chat in MAXQDA when analyzing their documents or codes. This definition implies a casual interaction, which is not the goal when conducting qualitative research (Paulus & Marone, 2024).
The second definition, “to exchange messages with somebody on the internet,” more closely aligns with what AI and ChatGPT proponents envision. However, calling it a “conversation” with AI or referring to the exchanged content as “messages” is also not entirely accurate. In MAXQDA, “chat” refers more to the interface type than the communication style or content. In an AI-based “chat,” humans input prompts, and the AI generates responses. This interaction is hierarchical in several ways, with humans guiding and AI reacting, and with humans ultimately judging whether or not the “conversation” meets the intended analytical objective.
Given these considerations, we propose using the term “prompt-assisted analysis” instead of “chatting with the data” when discussing MAXQDA’s chat feature. This terminology more accurately describes the process, as “prompt” refers to eliciting responses through questions or commands, while “analysis” overcomes the connotation of casual chatting.
Examples for Case-based and Theme-based Prompting
What can qualitative researchers achieve with this new prompt-assisted analysis? Currently (July 2024), AI Assist features two types of chats. One is the case-based “Chat with a Document” and the other one is the theme-based “Chat with a Code.” That is, either an entire document or a selection of previously coded segments are taken as a data basis to interact with this data in a prompt-assisted way.
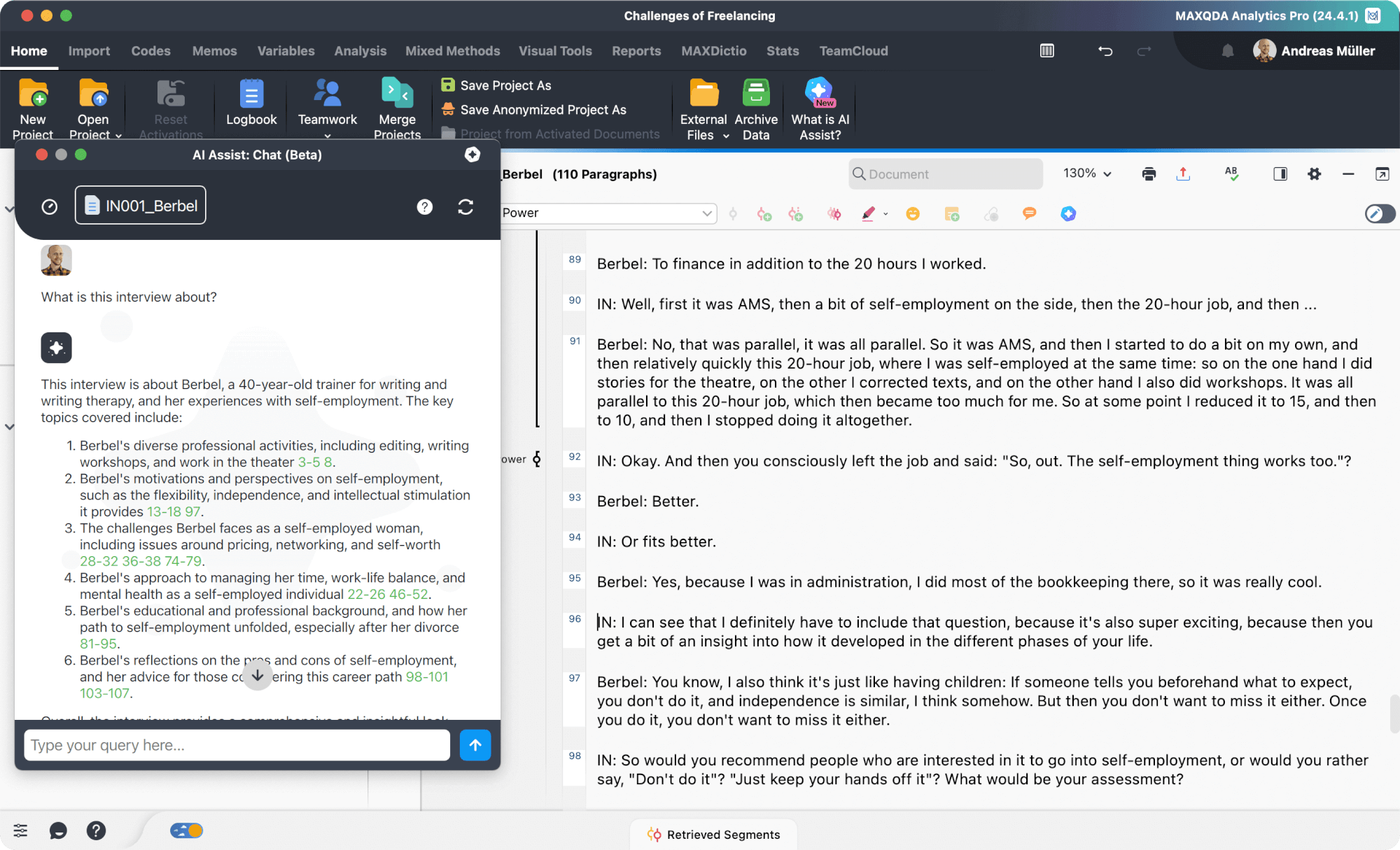
The “Chat with a Document” interface of MAXQDA’s AI Assist
This logic of working with either a case-based or a theme-based approach aligns well with the analytical techniques of established methods such as the “Case by Theme” matrix used to create a summary table (Kuckartz and Rädiker, 2023). In answering our research question, we often prioritize one perspective or alternate between them, for example, by reconstructing the internal logic and processes of a single case or by describing a theme across multiple cases.
Either way, currently, AI Assist supports textual data, including transcripts of interviews or focus groups, and published documents. However, image, audio, and video data are not yet supported. In the following, we will explore useful examples of prompt-assisted analysis, first focusing on case-based approaches, then on theme-based approaches.
Chat with a Document: Five Prompts for Case-Based Analysis
For case-based prompting, using the “Chat with a Document” feature of MAXQDA AI Assist, we distinguish between five different use cases: customizing summaries, developing a code system, identifying relevant data segments, receiving feedback, and assisting interpretation and analytical thinking.
First, let’s begin with a fundamental one: creating a document summary focused on one or more specific themes. After starting the chat for documents by right-clicking on the document name and selecting AI Assist > Chat with Document in the context menu, we enter the following prompt in the chat window:
Prompt 1: Customizing Document Summary
Summarize the transcript of the interview. Organize the summary in three sections:
1. [insert theme 1], 2. [insert theme 2], 3. [insert theme 3].
By specifying themes, we guide AI Assist to produce a summary that is more tailored than that generated by the “one-click” AI summary function that is also available in the context menu. This approach results in a focused and organized overview of the document’s content:
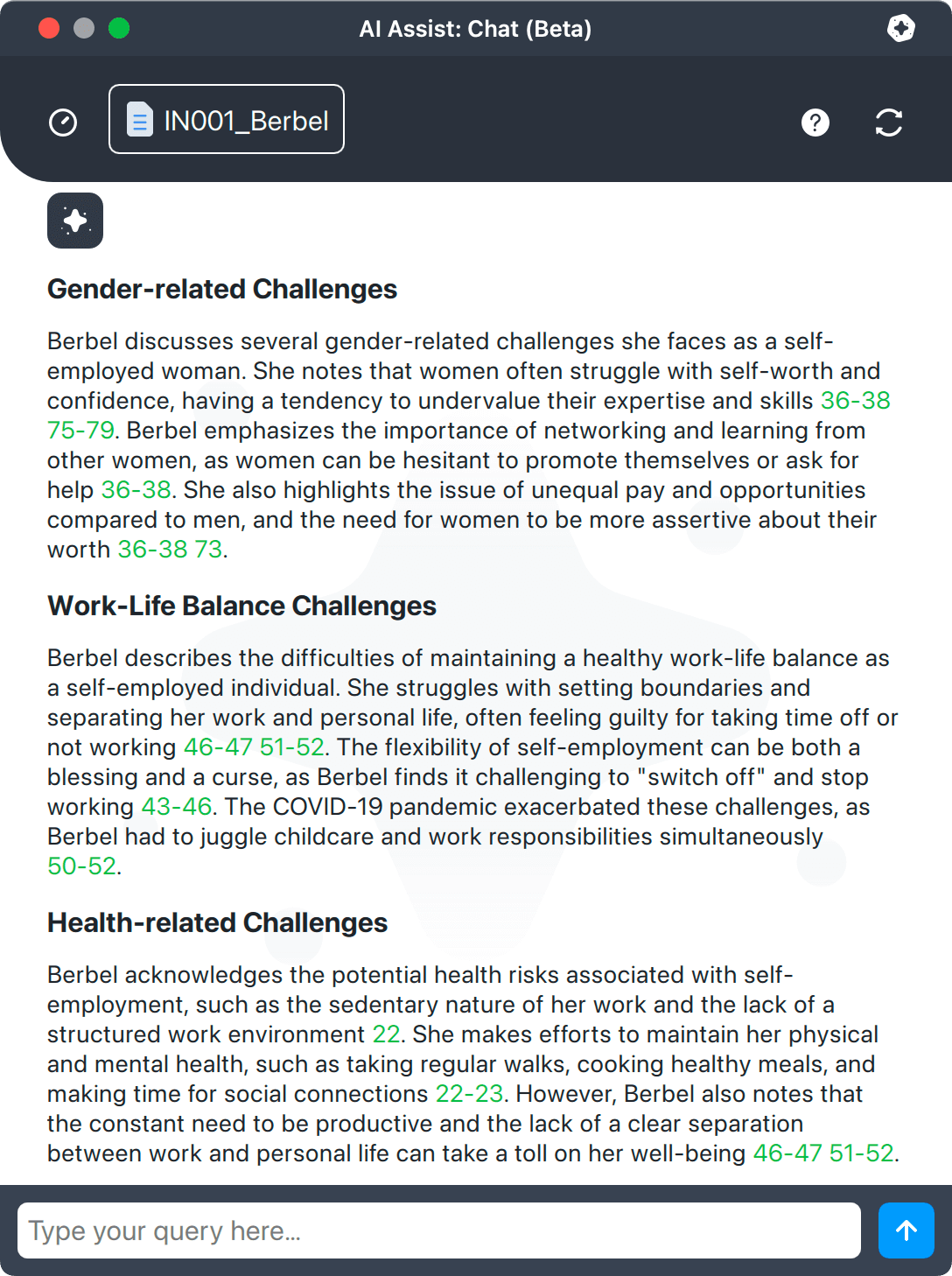
Example: Customized document summary
In this example, the AI creates a summary of the case considering only the specified themes and presenting them in the requested order. This approach offers a significant advantage over “one-click” AI summaries, which provide a general picture and may overemphasize sections of the data that are less relevant for the current analytical task.
Second, in coding-based analysis approaches, individual cases are often used to create inductive, data-driven, or emergent code systems. We can support this process with AI by using prompt-assisted analysis to create code suggestions based on a single document. For example, we can use the following prompt:
Prompt 2: Code Suggestions for a Document
Create a coding system that focuses on [insert your focus] with main and subcodes.
As we can see in the following screenshot, the AI response can inspire us with new ideas for a code system:
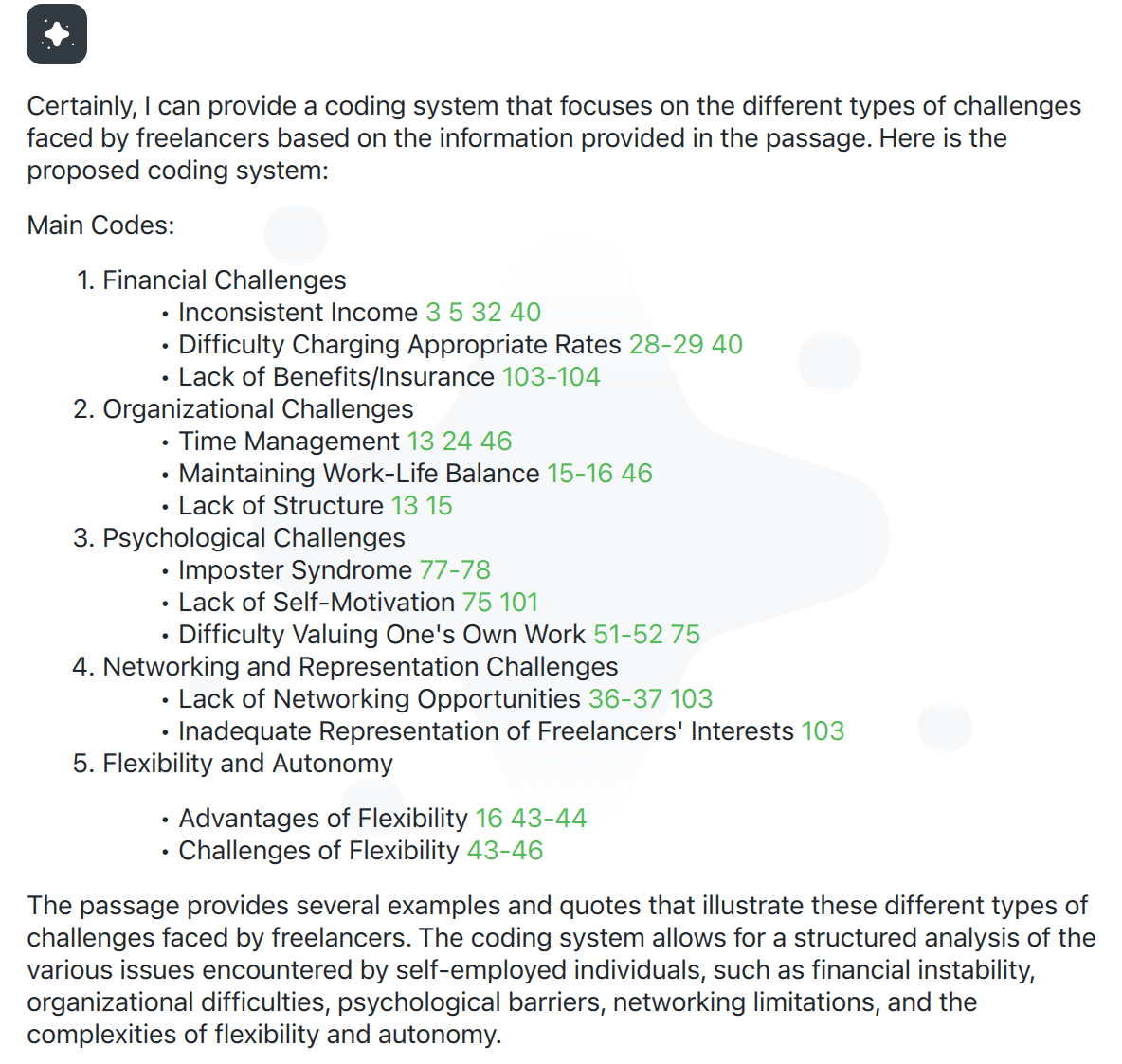
Example: Suggestions for codes based on a single case
By extending the prompt, we can further customize AI Assist’s output. We can specify what types of codes AI Assist should focus on, for example, by considering an existing theory or requesting code names in gerund form (the “ing” form”) as suggested by Charmaz (2014). Additionally, we can instruct it to follow a specific structure, such as differentiating at least two sub-levels and, by using follow-up questions, we can steer the AI to further differentiate or aggregate the suggested codes.
Third, we can utilize prompt-assisted analysis to identify specific data or information in the document. We could be interested in finding all locations, persons, organizations, or even animals mentioned in an interview. Of course, we could be looking for more abstract concepts like services offered by a freelancer. Here is a useful prompt for this purpose:
Prompt 3: Identifying Information in One Document on a Specific Topic
List all services that Fiona offers as part of her freelancing.
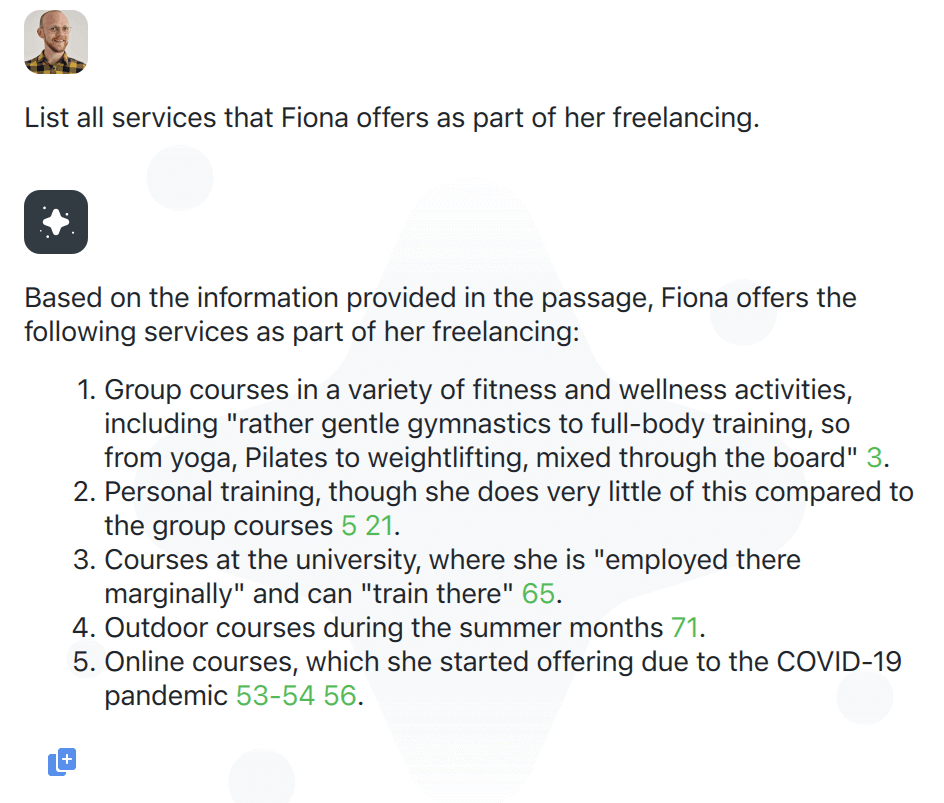
Example: Navigating the data
This approach becomes even more valuable as MAXQDA not only provides a response but also includes links to the corresponding passages in the original text. With one click, we can navigate to the relevant data. This feature offers a new way to retrieve and navigate our data and can even be helpful if we recall a specific passage from an interview but struggle to locate it quickly. However, it’s crucial to note, that quotations from the original text in the AI generated response may not always match the source word-for-word. It is comparable to asking a (good) student to remember a certain passage from a book. Therefore, it’s essential to verify direct quotations from the data against the original source.
Similarly, if the AI responds that no relevant segment was found, this does not guarantee its absence from the text. In such cases, it may be a good idea to use a follow-up question like “Please double-check if there are really no segments that could be seen or interpreted as …“. All in all, the use of prompts can be a quick and intuitive way to navigate the data, but the outputs must be treated with caution.
Fourth, feedback plays a key role in developing methodological skills for qualitative researchers. We can lever-age prompt-assisted analysis to receive valuable feedback on our written work and even on how we conducted an interview. When seeking feedback, it helps to ask for specific examples from the data in our prompt:
Prompt 4: Receiving Feedback on Interview Conduct
I am a researcher seeking to improve my interview techniques. Provide feedback on this interview, including specific examples from the text, and make suggestions on how I could have done it in a better way.
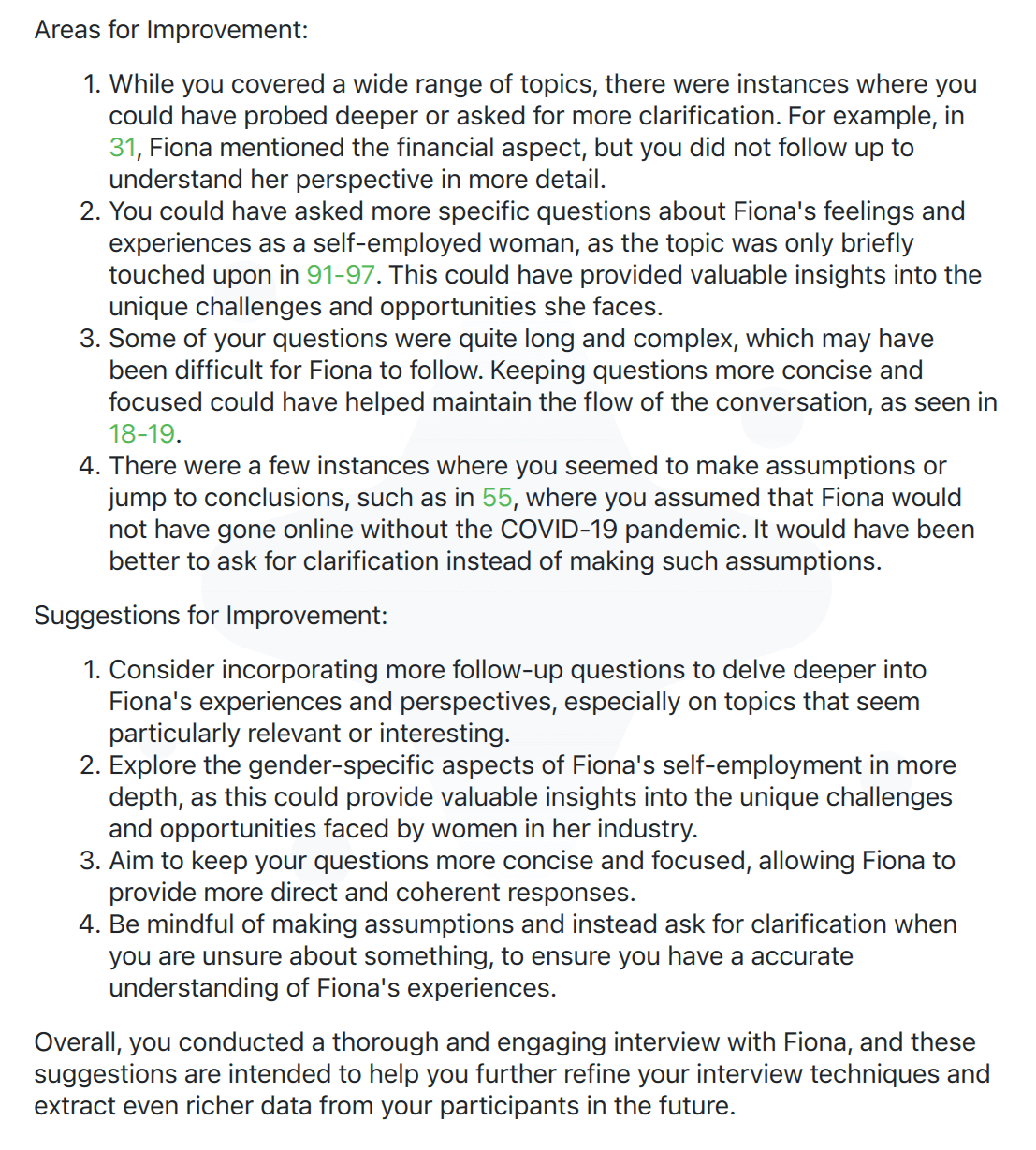
Example: Feedback for interview techniques
It is also possible to include instructions in the prompt to focus the feedback on specific aspects, like interview structure, question clarity, or interviewer neutrality. Using such prompts can help qualitative researchers to find relevant follow up questions, reconsider their role in an interview, or even point the finger at blind spots such as implicit racism, sexism, ableism, or other problematic behaviors that researchers may not be aware of.
The challenge lies in approaching the AI-generated “feedback” both openly and critically. As the recipient, you decide which suggestions to accept or discard. It is crucial to keep in mind that you are not engaging in a conversation with a “person” (and especially not an experienced researcher) but receive a plausible text based on probability scores from a language model. Human feedback from tutors with direct, real-world experience remains essential in this process. Nevertheless, it can be an interesting way to instantly challenge your own subjectivity even when it comes from an “unreliable methodologist” (the AI).
Fifth, we also see major potential in using prompt-assisted analysis as a powerful tool to strengthen and inspire our analytical thinking. Let’s look, for example, at the following prompt:
Prompt 5: Identifying Contradictions in an Interview
Identify where the interviewee contradicts themselves in their statements.
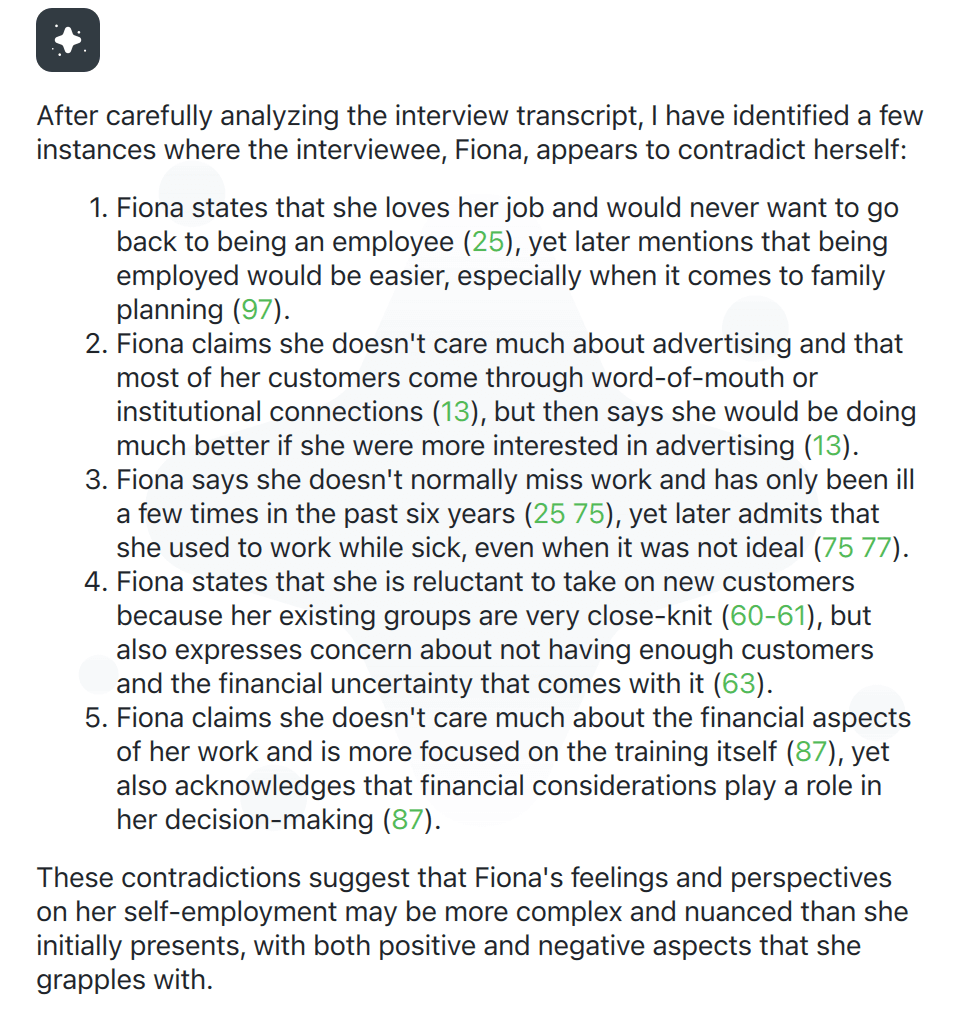
Example: Assisting analytical thinking and interpretation
While the output may not reveal strong contradictions, it highlights subtle ambiguous feelings regarding pros and cons of freelancing. Prompts such as “What story is the person telling through the conducted interview?” or “As whom does this person see themselves?” can help us deepen our interpretation of an interview and support our conceptual and analytical work. By asking the right questions (using good prompts), researchers can find new angles or insights for existing data and compare their own analytical work with an alternative version from an AI.
As we have shown, case-based prompting can enhance the analysis beyond merely creating simple summaries. In the next section, we will turn to a more thematic approach.
Chat with a Code: Five Prompts for Theme-Based Analysis
Chat with a Code: Five Prompts for Theme-Based Analysis In the following examples, our prompt-assisted interaction focuses on already coded data rather than entire documents or cases. This requires that codes have been created and applied to our data by either manual methods or text search and autocoding. Unlike the case-oriented approach, the quality of the prompt-assisted analysis depends heavily on how the coding was conducted. Common methodological question such as “How large should my coded segments be?” or “What data should I (not) code?” are important here. AI Assist will produce better results if the coded segments contain enough context to answer the queries asked in the chat. If the chat responses are unsatisfactory, it is often a good idea to review the underlying coding by examining the coded segments in the “Retrieved Segments” window.
For theme-based analysis, our five prompts follow similar use cases as presented for case-based analysis above: customizing code summaries, suggesting subcodes, retrieving coded segments, feedback on the coding process, and assisting interpretation and analytical thinking. The MAXQDA chat for coded segments can be opened at several locations in MAXQDA:
- By right-clicking a code name and selecting AI Assist > Chat with Coded Segments of This Code (all coded segments of the code will be considered).
- In the “Retrieved Segments” window, in the Smart Coding Tool, or in the Categorize Survey Data workspace using the AI Assist icon (only the currently listed coded segments will be considered).
First, we can create customized code summaries. Compared to the case-based approach, narrowing down on a certain theme is not of such relevance, as the coding already includes a theme-based selection process. Instead, we can focus on requesting a certain output structure, for example:
Prompt 6: Customizing Code Summary
Summarize the coded segments. In a first section, focus on the common ground shared by most interview participants. In a second section, highlight the unique or divergent statements and opinions.
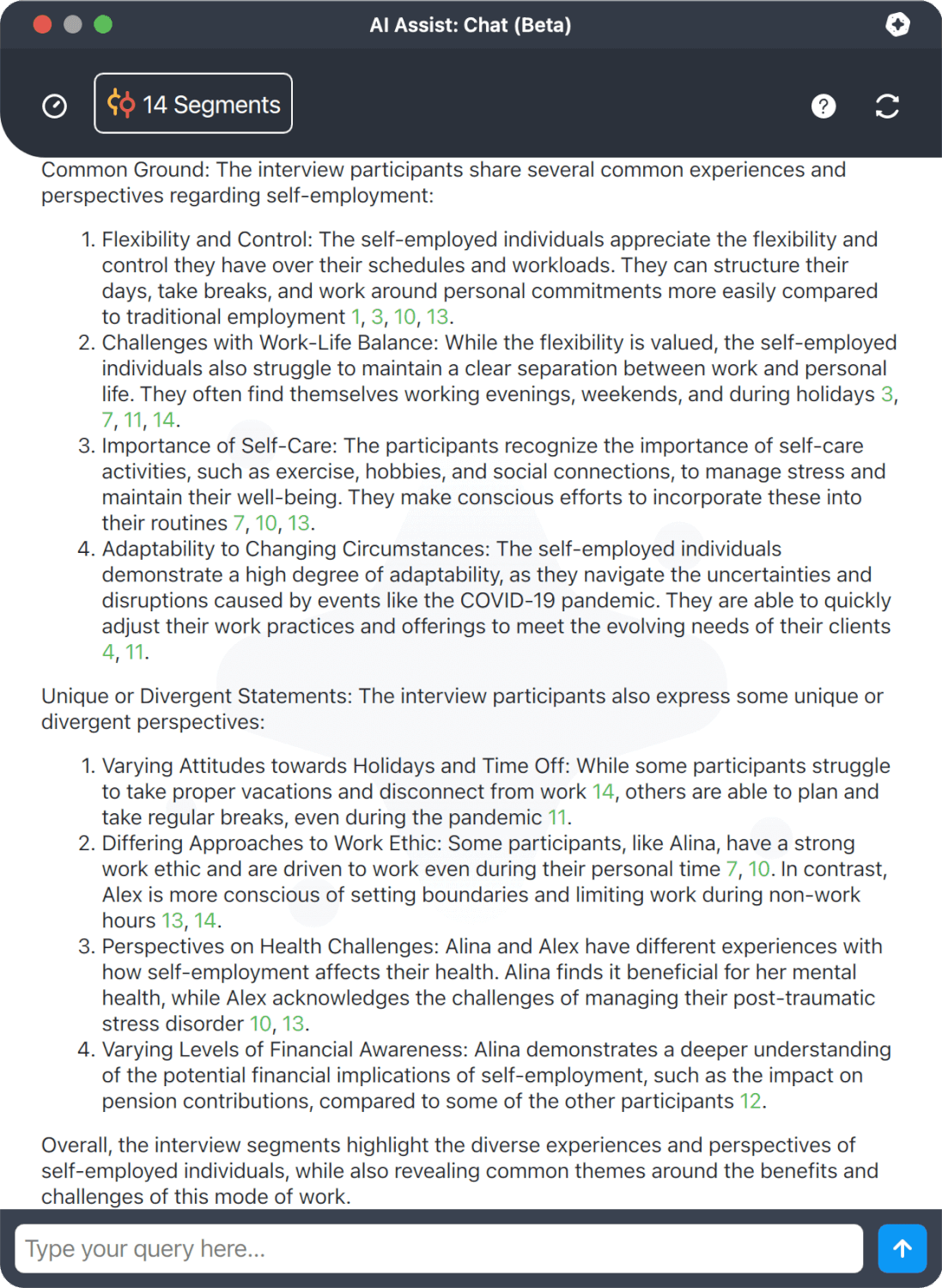
Example: Customized code summary
In the example, the prompt is used to first describe the “common ground” of the research participants on the theme covered with the code. What do they agree on? Secondly, individual opinions are summarized. Please note that the chat response contains the pseudonyms of the interviewees, since they were used as document names and within the documents to identify the speakers. Again, we can check the accuracy of the responses by following the clickable references, because for chats with coded segments, each of the segments is given a unique number.
As a second use case, we may utilize prompt-assisted analysis to create ideas for our code system. Here, we can use this approach to further differentiate an abstract topic or theme into more specific subcodes. For example, we can prompt for suggestions for the code “negotiating power” and require the AI to split it into “micro” and “macro” aspects:
Prompt 7: Creating Custom Subcode Suggestions
Make suggestions for subcodes to further divide the code “negotiating power”. Split the suggested sub-groups into two groups: 1) big-picture “macro” factors that impact negotiating power and 2) individual “micro” factors that impact negotiating power.
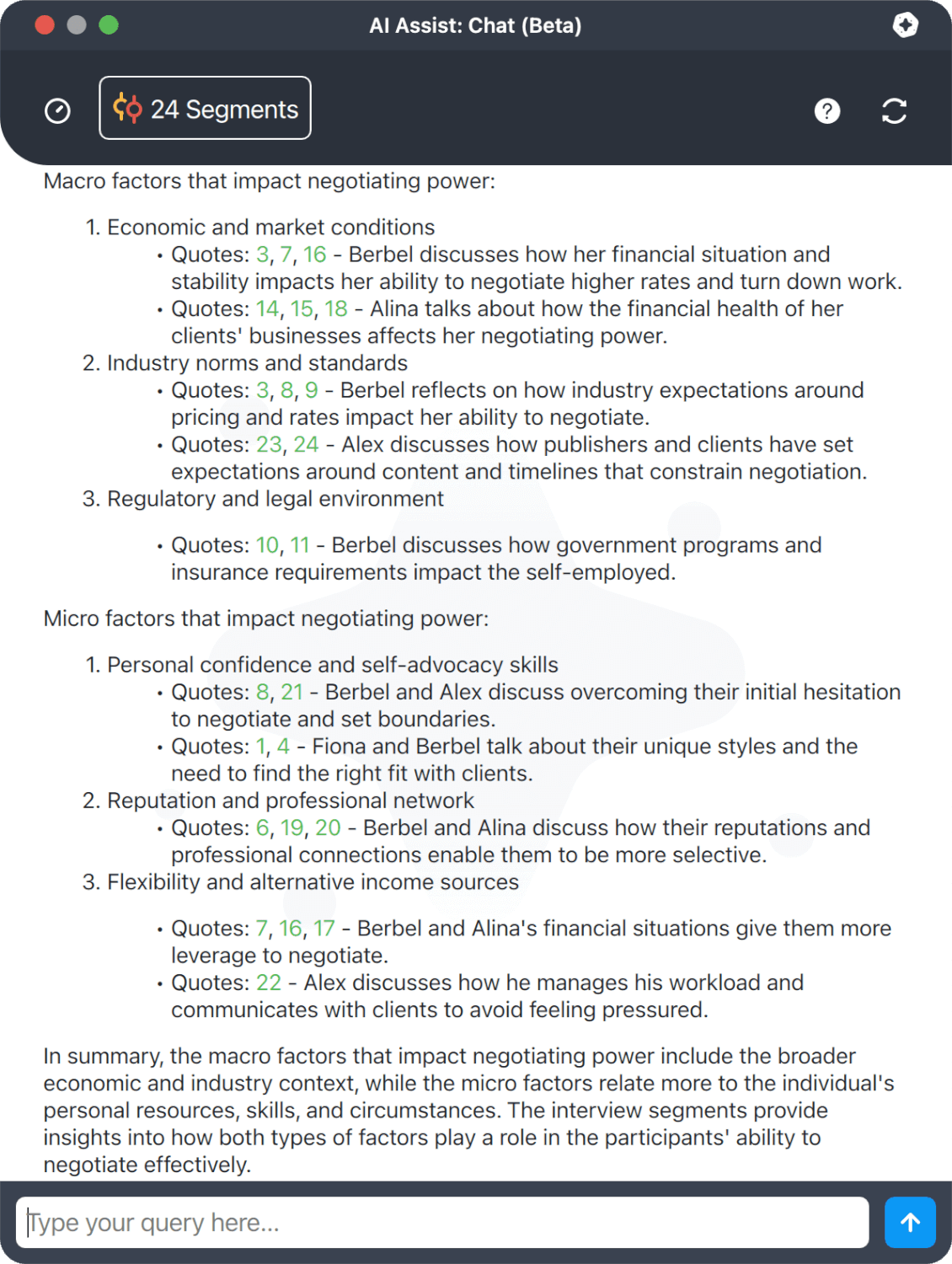
Example: Customized code suggestions
This may help us move beyond simple descriptive codes by introducing a more analytical and theoretical perspective into our subcode layer. We will also receive many examples from our data thanks to the references which can be very helpful for developing empirically grounded subcodes.
As a third use case, we can employ a prompt to identify one or more segments, which aids in navigating our data:
Prompt 8: Identifying Relevant Segments
Help me find the segment in the data where [insert your criteria].
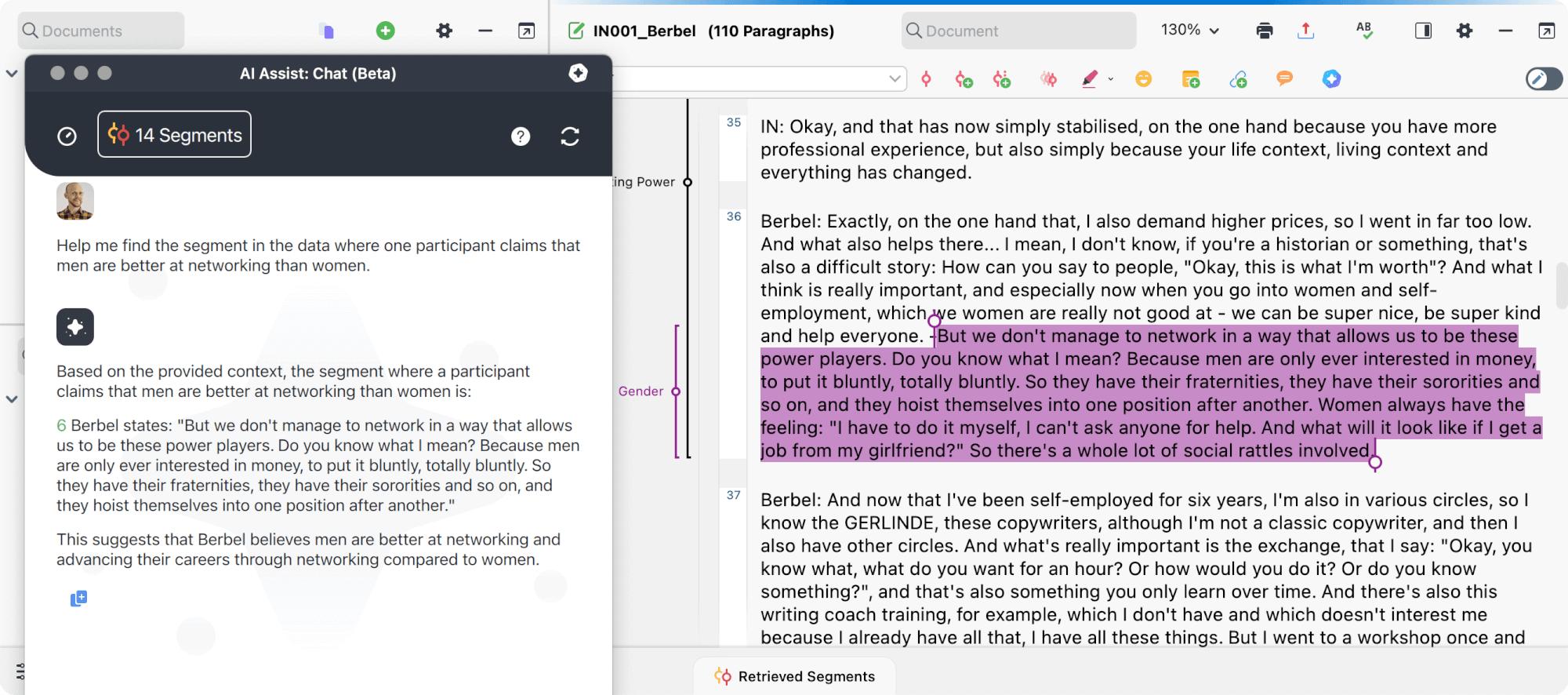
Example: Retrieving Relevant Quotes
This can be a very useful and quick search, as MAXQDA’s AI Assist references the number of the coded segment, and a single click allows us to check the results. This is less error prone, because the AI only needs to find and reference and thus pick from existing segments. But keep in mind that direct quotations in the AI response should not be considered reliable, instead, we always recommend checking the identified source and quoting directly from the original data.
Fourth, we may use prompt-assisted analysis to evaluate the quality of our coding and code definitions:
Prompt 9: Quality Check of the Coding and Improving the Definition
The segments have been coded with a code labeled as [insert code name]. I defined the code as follows: [in-sert code definition].
Identify segments that do not match this definition.
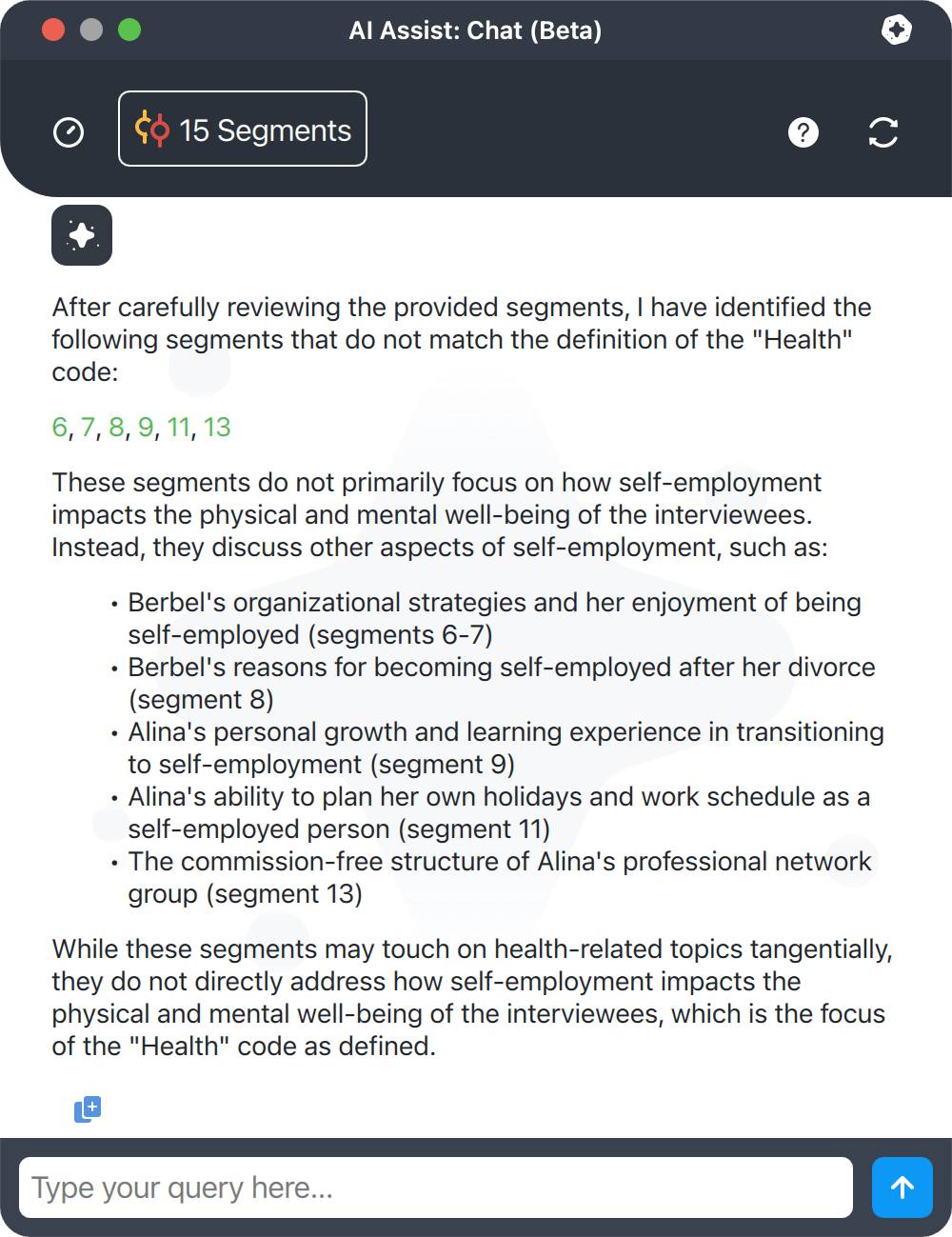
Example: Checking coding quality
This prompt helps us to identify incorrectly coded segments that may not belong to a certain code, possibly due to mistake or code merging. It helps to ensure that our codes actually contain the data we think they do. Again, the references make it easy to double-check the identified outliers. Furthermore, it’s often beneficial to use AI for improving the code definitions, for instance, using a follow-up prompt like “Considering the coded segments, suggest improvements for my code definition”.
Finally, prompt-assisted analysis of coded segments can support our interpretation and analytical tasks. Again, we have an example with segments coded with “negotiation power:”
Prompt 10: Grouping Coded Segments Based on a Certain Criterion
I am interested in whether the research participants consider their own negotiation power strong or weak. Make suggestions for grouping the statements by the level of negotiation power and list the segments for each group.
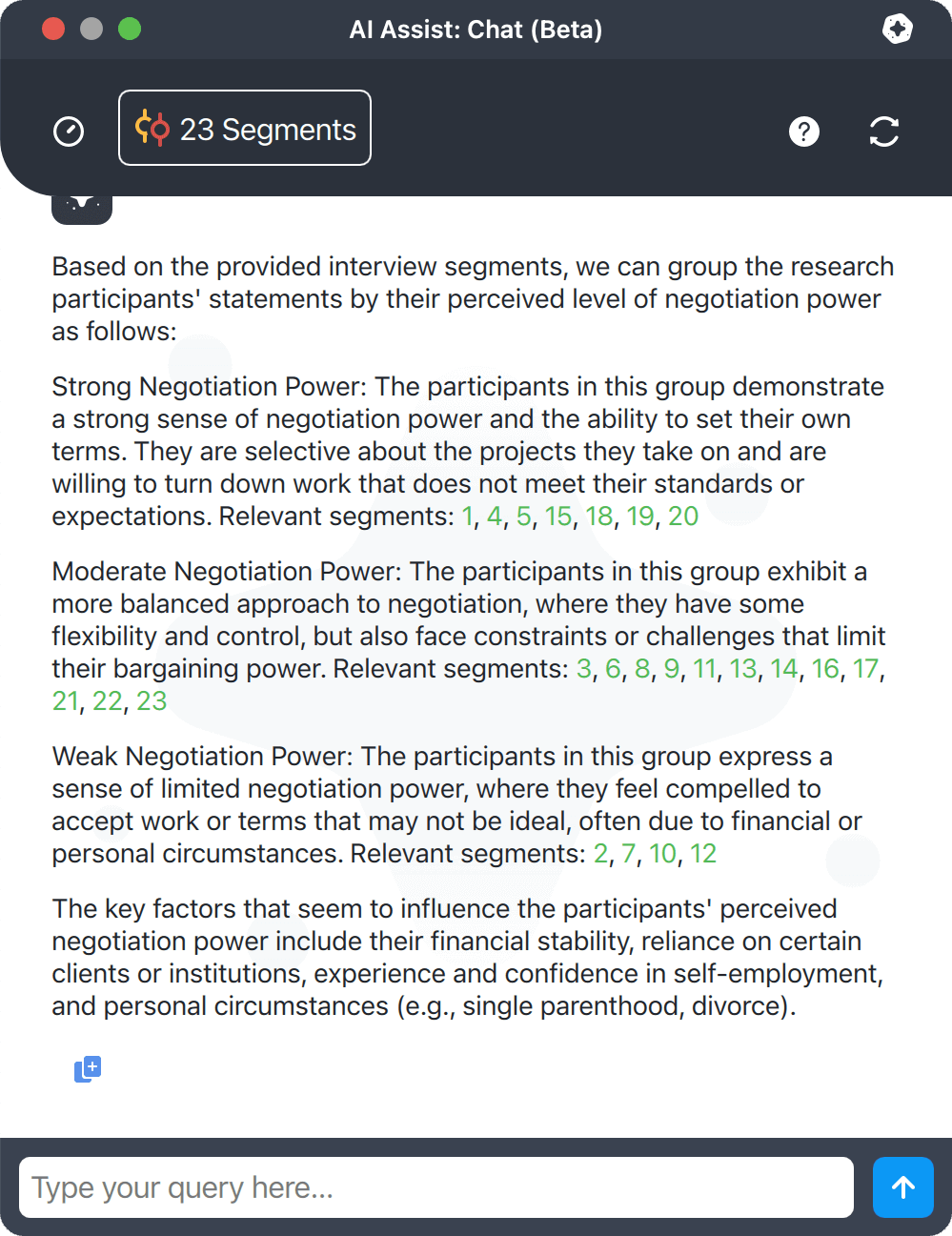
Example: Grouping coded segments based on a certain criterion
In the example, the AI identified several different degrees of negotiating power in the data along with explanations and references to the segments. Responses like this may support analytical tasks, such as developing appropriate scales for a typology (Kuckartz & Rädiker, 2023).
Overview of the Use Cases
The following table sums up the five use cases for both case-based and theme-based prompt-assisted analysis presented in this blog post. For each use case, we have listed one possible analytical result:
Case-based | Theme-based | |
---|---|---|
Summarizing | Theme-focused case summary | Common themes and unique perspectives in a summary |
Developing codes | Coding frame for a specific case | Subcodes further differentiating a main code |
Navigating data | Specific sections within a document | Specific coded segments |
Receiving feedback | Ideas for improving the interview technique | Ideas for improving code assignments and code definitions |
Assisting interpretation and analytical thinking | Contradictory opinions and statements within one interview | Scaled grouping of similar interviewees’ responses |
These use cases are of course anything but exhaustive. We are sure that our readers may soon wish to add several more, yet the presented use cases reflect typical research needs and provide a good starting point for applying prompt-assisted analysis.
Additional Tips and Conclusions
The listed use cases and prompts offer valuable insights into the capabilities of MAXQDA AI Assist’s chat feature. We hope they inspire further researchers to broaden the scope of prompt assisted analysis beyond simple writing of summaries. To maximize their potential, we suggest considering the following best practices when using the chat:
- Simple follow-up questions such as “elaborate further” or “explain aspect x in more depth” make optimum use of the chat’s capability to consider the previous conversation.
- If you don’t get the expected results or if you begin a new theme, reset the chat to prevent previous conversations from influencing the latest answers.
- To improve answer quality, try extending a prompt by asking for reasoning: “Justify your answer!” or “Provide detailed reasons in your answer!”
- It is also possible to request tables or ask for specific formatting of the output.
Prompt-assisted analysis with an AI “intermediary” between the researcher and their data offers many new analytical techniques. For sure, these techniques can be utilized within the framework of established qualitative research methods, and as our examples illustrate, they have great potential to enhance the established methods.
Currently, there’s limited literature on how to effectively incorporate AI and prompt-assisted analysis techniques into established methods, with some exceptions like Kuckartz & Rädiker’s work on qualitative content analysis (in press). With this blog post, we hope to have contributed to fill this gap and give some orientation.
Right now, we are at the beginning of an exciting journey in research methodology, where innovative uses of AI in qualitative research are constantly evolving. For this reason, we eagerly anticipate your feedback and experiences with prompt-assisted analysis. Please share your thoughts with us at andre-as@muellermixedmethods.com and raediker@methoden-expertise.de.
References
Kuckartz, U., & Rädiker, S. (2023). Qualitative content analysis: Methods, practice and using software (2nd ed.). SAGE. https://qca-method.net
Kuckartz & Rädiker (in press). Qualitative Inhaltsanalyse. Methoden, Praxis, Umsetzung mit Software und künstlicher Intelligenz (6. ed.). Beltz Juventa. https://qualitativeinhaltsanalyse.de
Paulus, T. M., & Marone, V. (2024). “In minutes instead of weeks”: Discursive constructions of generative AI and qualitative data analysis. Qualitative Inquiry. https://doi.org/10.1177/10778004241250065
Authors
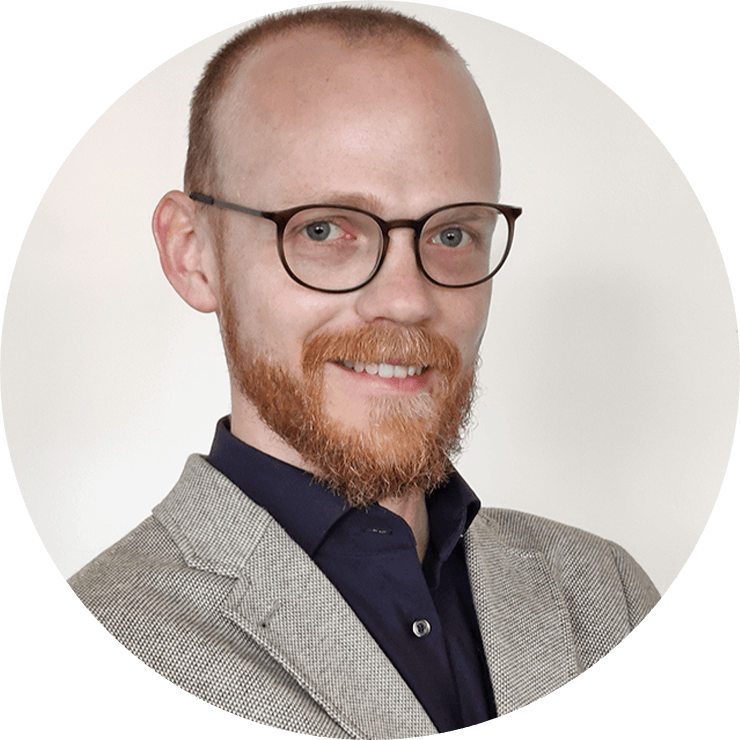
Andreas Müller has been a professional MAXQDA trainer for over seven years, and is a research and methods coach and contractual researcher. He is experienced in a wide array of qualitative methods and works with clients from diverse academic disciplines, including healthcare, educational sciences, and economics. Andreas specializes in the analysis of qualitative text and video data, as well as mixed methods data.
www.muellermixedmethods.com
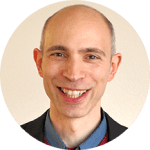
Stefan Rädiker is a consultant and trainer for research methods and evaluation. He holds a PhD in Educational Sciences and his research focuses on computer-assisted analysis of qualitative and mixed methods data. He has worked with MAXQDA for more than 20 years and supports the VERBI team with his methodological expertise in the development of the MAXQDA software.
www.methoden-expertise.de
Citation (in APA format):
Müller, A. & Rädiker, S. (2024, August 12). “Chatting” With Your Data: 10 Prompts for Analyzing Interviews With MAXQDA’s AI Assist. MAXQDA Research Blog. https://www.maxqda.com/blogpost/chatting-with-your-data-10-prompts-for-analyzing-interviews-with-maxqda-ai-assist