Documentation of the Symposium about the opportunities and challenges of AI in (qualitative) research at the MQIC 2024, February 29, in Berlin
Participants
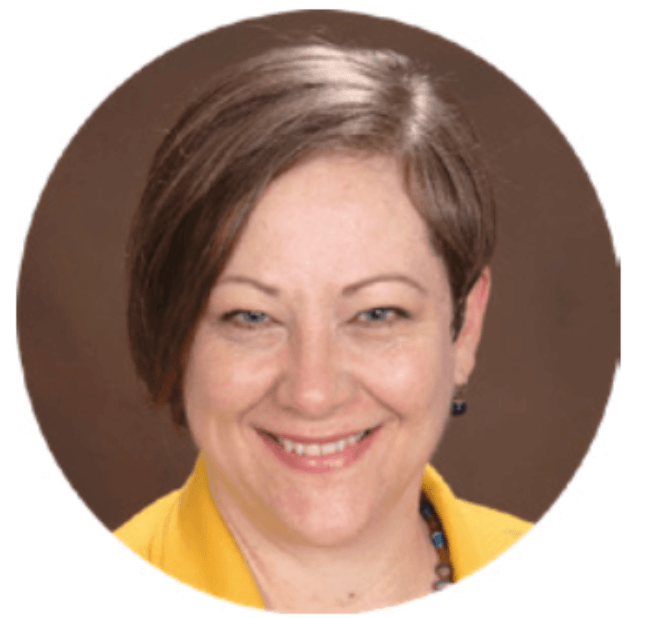
Dr Karen Andes
Professor at Brown University
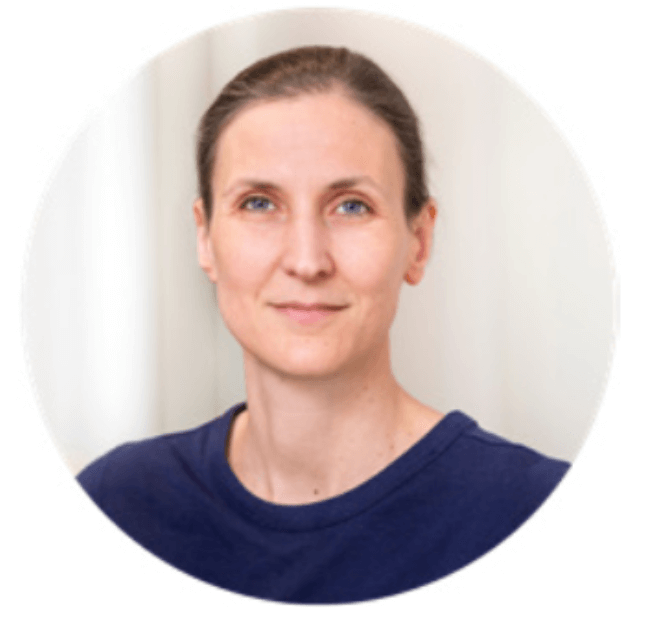
Julia Gerson
Chief Product Manager of MAXQDA
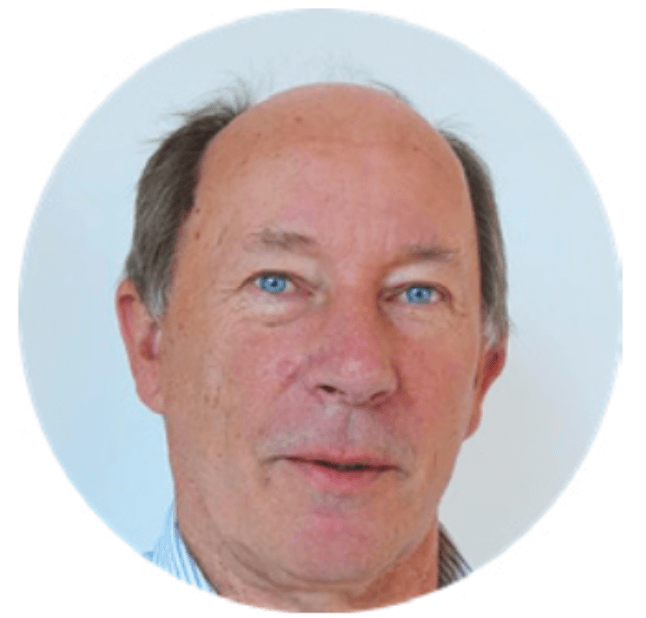
Dr Udo Kuckartz
Professor Emeritus at Philipps University Marburg
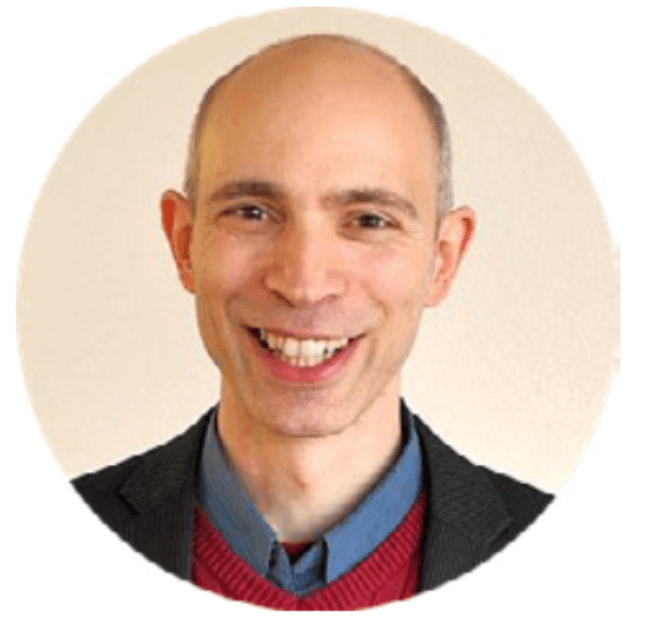
Dr Stefan Rädiker
Research Consultant, research-coaching.de
Moderator
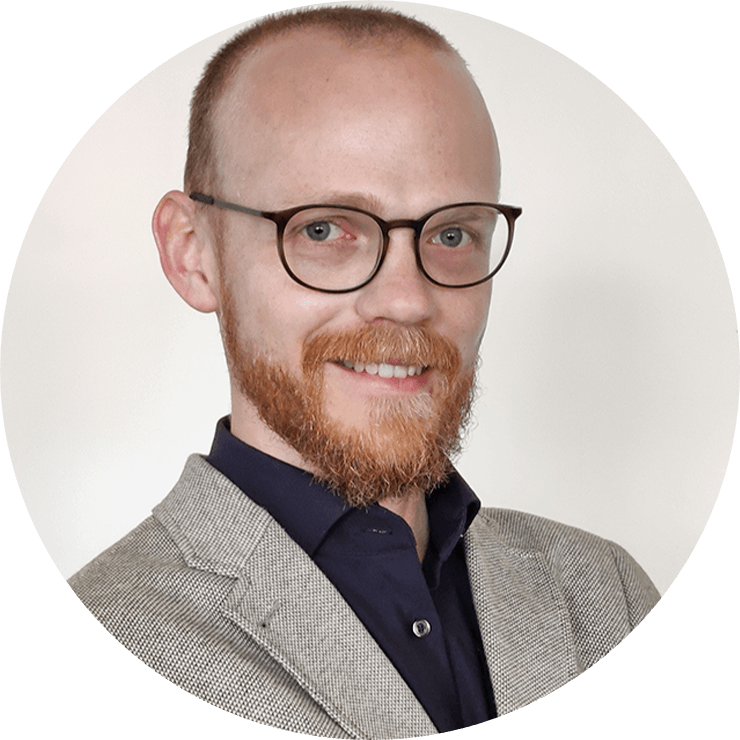
Andreas Müller
Research Consultant, muellermixedmethods.com
Introduction
At this year’s MAXQDA International Conference (MQIC), an interactive symposium discussion featuring four participants delved into the opportunities and challenges of AI in research – more specifically AI in qualitative research. The discussion also incorporated questions and comments from the audience.
Prior to the event, the four participants were instructed to prepare statements in response to the following three key questions:
- What opportunities do you see in your area of research and work when using AI?
- What challenges are you facing in your area of research and work because of AI?
- Regarding the implementation of AI Assist within MAXQDA:
– How do we make sense of the analytical output produced by AI Assist? or
– How does the workflow change when analyzing data? – now and in the future.
To visually support their perspectives, participants created or chose images that aligned with their statements for each question. The symposium’s discussion followed a structured format for each key question, starting with each participant presenting their image and articulating their viewpoint within a 90–120 second timeframe. This was followed by participants commenting on each other’s images and assertions, subsequently opening the floor to audience participation.
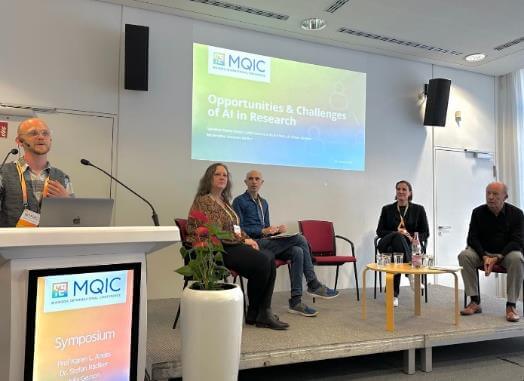
This document summarizes the key arguments, insights, and discussion points raised during the symposium, organized around the three questions, and followed by some concluding remarks. Please note that the views expressed in the discussion do not necessarily reflect the opinions of the panelists.
1) Opportunities of AI in Research
Stefan Rädiker
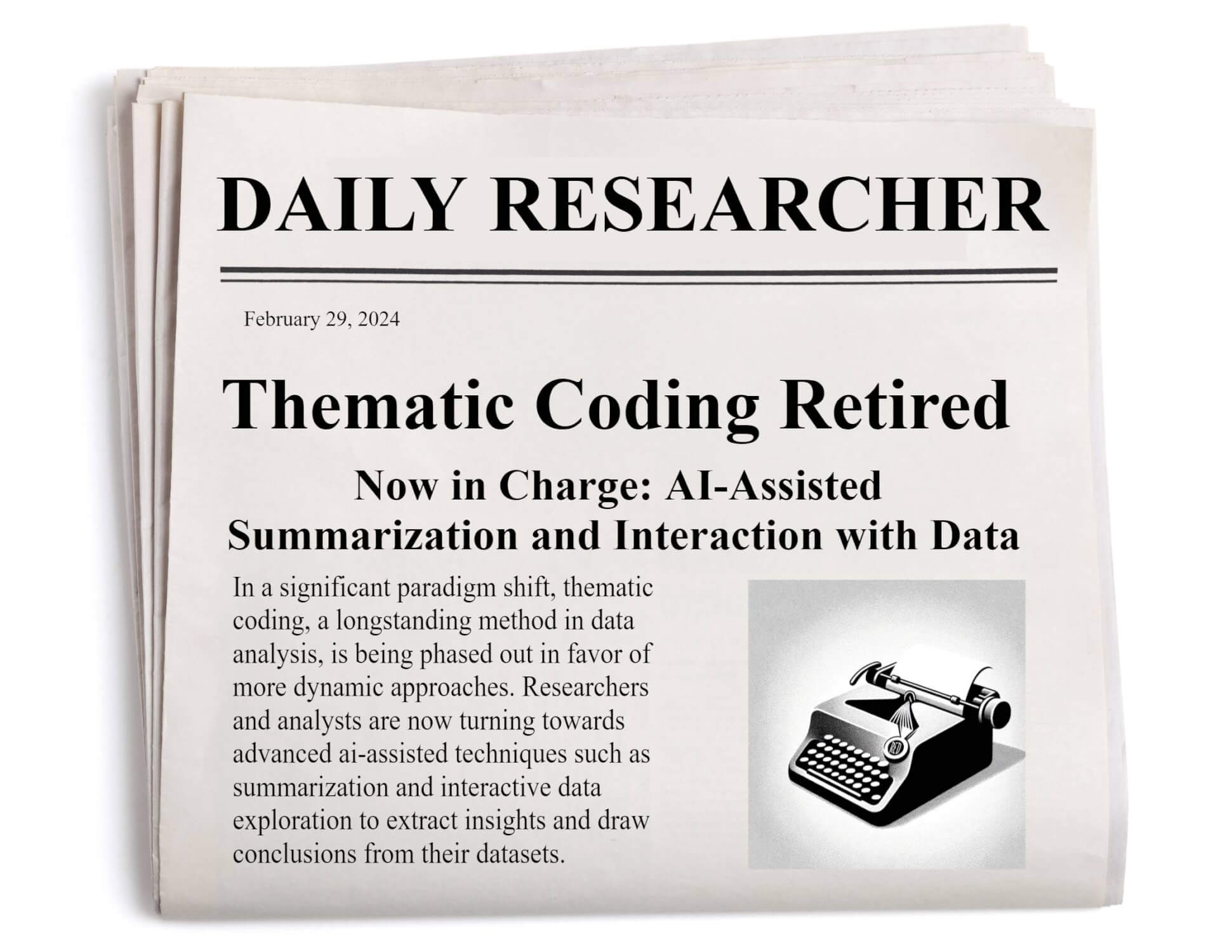
One of the opportunities of AI in research is the emergence of new promising analysis methods. Some researchers have already argued that ai-assisted summarization and, particularly, interacting with the data will be more important than current approaches of coding the data. I think this may be true for thematic coding, since we see that it is already possible to identify and summarize topics within the data skipping the coding process.
After considering to show an image with a tombstone and the day of death of thematic coding, I chose the less provocative analogy of retirement, because I am still convinced that the ideas of coding and thematic coding will be alive and they have a long and deserved tradition for a reason. However, I see the opportunity that thematic coding will be transformed into new ways of doing thematic-oriented analysis.
Karen Andes
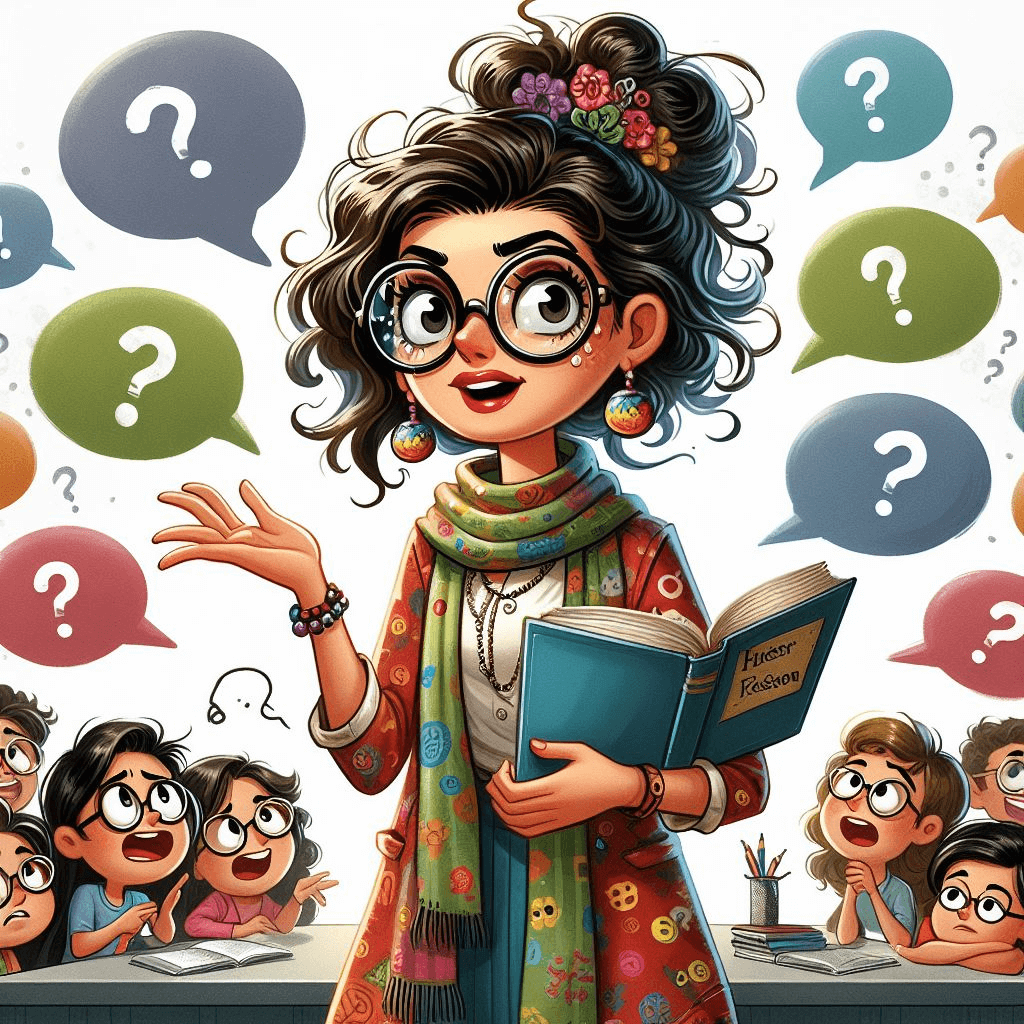
I brought you a picture of the naive professor. Of course, the first thing I did when I was asked to join the panel was, I asked ChatGPT to develop a speech for me and I thought it was very interesting that it touched on inductive and deductive coding, but I’m not going to give you that. I was skeptical at first, I’ll be really honest. But as I’ve come to see how it’s been implemented in MAXQDA, I’ve been really excited about the opportunities that it presents, especially in terms of students. I’m always working with students who want to know if they’re doing things right and they want to have assignments. When I ask them to do a thematic analysis, they want to see what the assignment looks like from somebody who’s done it well once before. And I see there may be opportunities now to have students kind of pin themselves against this, this partner in research that may not do everything quite how we want it to, but gives them a point of departure and gives them a point of comparison. That and I’ll talk about this a little bit more in a bit that may actually mimic teamwork.
Julia Gerson
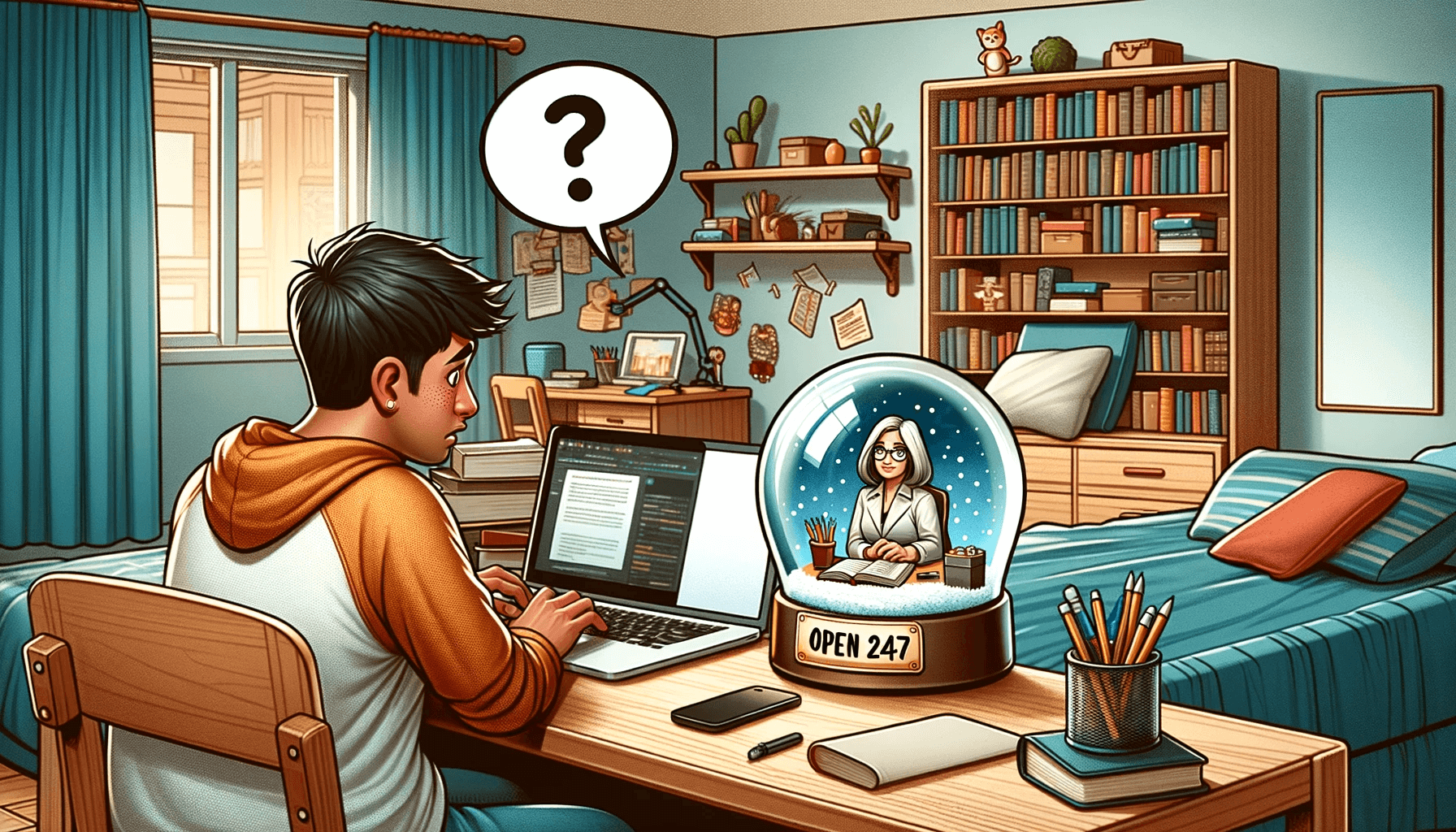
My image portrays AI as a 24/7 assistance in a learning environment. It shows a student in a dorm room working on a laptop, with a snow globe sitting on the desk. The snow globe contains a figure symbolizing a knowledgeable guide or teacher, illustrating the role of AI. This AI guide may not be an expert on the very specific topic the student is currently working on, but it has a general textbook knowledge about every imaginable topic, including research and MAXQDA-related topics.
The student can ask the AI to explain Grounded Theory as if they were talking to a ten-year-old. This results in a fun answer that includes Lego bricks and being a detective trying to fit bricks together and building different models.
I also use this capacity of AI in my role as a product manager to receive general tips while planning meetings or speeches or getting feedback on design mockups.
While AI might not replace specialized expertise, its wide-ranging knowledge and constant availability offer substantial support. An example of AI’s practical use is in the MAXQDA manual, where a chatbot provides instant assistance, demonstrating AI’s value in providing immediate support.
In conclusion, AI serves as a significant tool in both educational and professional settings with its constant availability to provide valuable feedback, answer questions, and explain concepts.
Udo Kuckartz
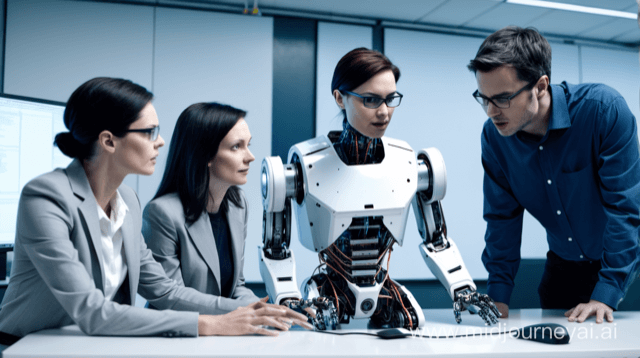
The first image I brought with me shows the possible future of social research. A research team at work, supported by AI, represented here by a human-like robot. AI offers great opportunities for social science research, it can increase efficiency and productivity and automate many tasks in the research process, for example transcription. AI can also help with writing and presenting. Researchers are relieved of operational tasks such as transcribing and coding. AI allows us to focus on intensive analysis and answering our research questions. In general, data analysis will become more dynamic and interactive.
Today’s situation reminds me of the 1980s. In 1984, exactly 40 years ago, I began to explore the possibilities of computer-assisted analysis of qualitative data and to develop appropriate software. It seemed clear to me that the use of software would greatly change the process of qualitative data analysis. At that time, there was strong resistance to the use of computers, for example from Barney Glaser, the co-author of grounded theory. It took a relatively long time for QDA software to become the standard for qualitative data analysis. Something similar will probably happen with AI. Perhaps everything is a little faster today. In any case, my conclusion is that AI tools offer a great opportunity to improve our research techniques.
Discussion of Panelists
- Using AI Assist in research can save time, but the key question is what we do with the time we save. Rapid analysis may be helpful for some tasks and areas, such as evaluation and strategic foresight, but using AI to improve efficiency also allows us to skip tedious tasks like transcription and move on to more analytical tasks. It also allows us to be challenged by the AI results and deepen our analysis. (Stefan Rädiker)
- AI Assist should not be thought of as a human. It has specific capabilities, such as a huge textual memory to read and “understand” a book I have written in seconds – while I, as a human, cannot remember all the details I have written. This allows me to ask questions about my own book. (Stefan Rädiker)
- Both teachers and students have questions about AI Assist and it is important to be able to go through the process of discovery with AI Assist in MAXQDA to evaluate how well it works. (Julia Gerson, Karen Andes)
- In the theory of the sociologist Ulrich Beck, the second stage of modernity, which began in the 1970s, is marked by the rationalization of culture and private life. This has resulted in a culture where people “score” potential partners and compare them, leading to frequent divorce and a focus on individualism. Now with AI, we may be entering a third stage of modernity, where individuals are increasingly isolated and have more free time. But with what do they fill their time? With entertainment such as watching Netflix? (Udo Kuckartz)
- Generative AI can sometimes produce unexpected results when creating content, particularly images. These surprises can act as “light bulb” moments, helping to clarify research problems and revealing new insights. (Stefan Rädiker)
Discussion with Audience
- AI-generated images can perpetuate stereotypes and biases, such as depicting female students seeking help from male assistants. These representations can influence how we perceive society. But the apparent bias in AI-generated images may simply reflect specific user prompts, rather than inherent bias within the AI system (as it was the case with Karen Andes asking for a female professor with a question mark). (Audience member, Karen Andes)
- Although AI may be biased, similar biases exist in human behavior. The promising option of generative AI is that we can go back and forth when, for example, prompting to create an image – and by using this technique we also play a role in shaping biases. (Audience member)
- AI in research has an assistant role taking over tedious tasks for researchers. (Audience member)
- Do we need to investigate how to respect AI as entities with rights and (working) regulations to ensure respect for the AI? What if AI were to unionize in the future? (Audience member)
- AI should not be humified and should not be viewed as having rights due its algorithmic nature. ChatGPT is a technical tool for predicting words and simulating human interaction. We need to be cautious against over-humanizing AI and emphasize the importance of understanding its limitations. (Andreas Müller)
- As teachers for qualitative research, we need to reflect on how to utilize AI to foster critical thinking and autonomous learning in the next generation of qualitative researchers. For example, we can create exercises that will encourage students to interact with AI in meaningful ways and help them realize that AI doesn’t have empathy or feel the need (as we do) to answer questions. (Audience member, Karen Andes)
- When AI transcribes for us, we may lose some of the important interaction with the data. Therefore, it can be helpful to transcribe parts of the data on our own. However, checking the transcripts for accuracy, especially those of interviews that we did not conduct ourselves, helps us to become familiar with the data and begin to analyze it. (Audience member, Stefan Rädiker)
2) Challenges of AI in Research
Julia Gerson
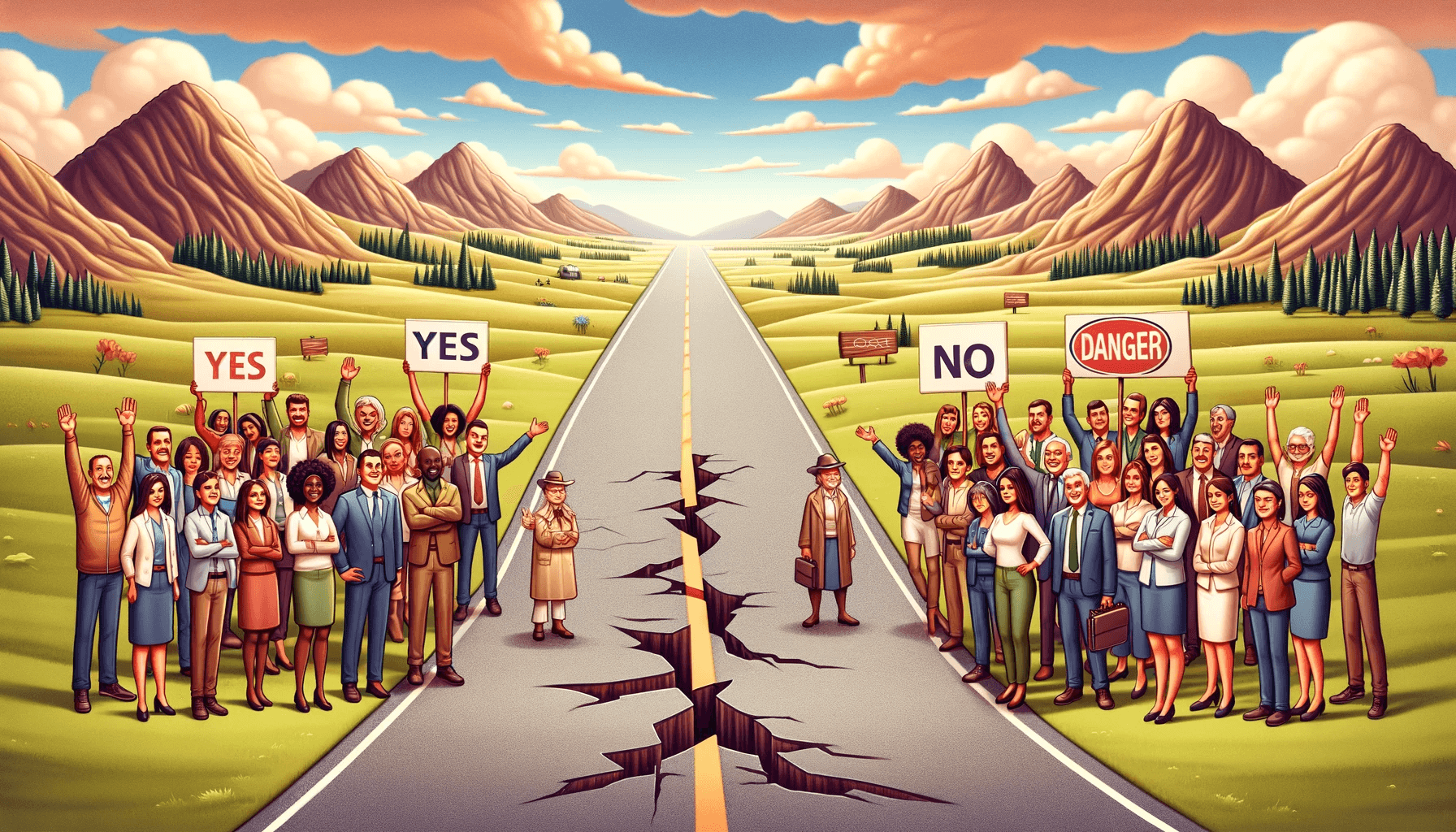
This image is guided from my point of view as the MAXQDA product manager and the challenges we’ve been facing over the last year since OpenAI released ChatGPT.
In this image you can see a road, which illustrates the MAXQDA roadmap, which is the term we use in software development to plan out which features we want to work on next or in the future. On the side of the road, you can see two groups of users. In this image, they are equally sized groups, but actually the “enthusiastic” group should be larger. Both groups are voicing their opinions, one of them holding positive “Yes” signs. This group wants to work with AI features in MAXQDA and would like to have those available as fast as possible.
And on the other hand, there is a group of users who are skeptical and concerned. They are holding “No” and “Danger” signs. They are sending us questions about privacy. They don’t want AI to interfere with their research. They are working for organizations that cannot purchase a software if it contains AI cloud tools.
The challenge we had to navigate was to listen and understand both user groups and find solutions that fulfill both of those needs. For example, by implementing AI Assist as an optional add-on and ensuring to fulfill the highest security standards.
You could expand the horizon not just to MAXQDA but to qualitative research in general, where you also have both types of voices and reactions regarding the usage of AI in research. I am curious to see how the method or research community will resolve the same issue.
Udo Kuckartz
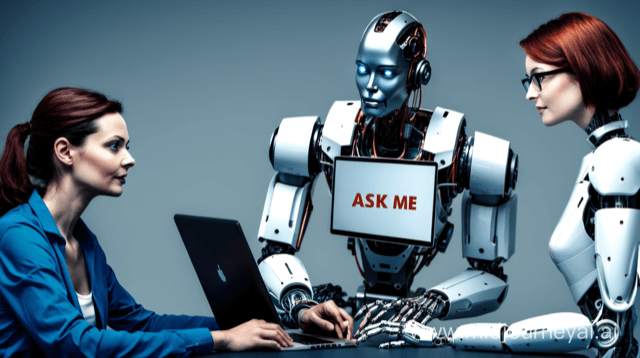
This image deals not only with the challenges for my own research, but also with general challenges that affect us all. Like the first image, I created this image using the AI tool Midjourney. What do we see here in the photo – or what do we think we see here? On the left is a person, a researcher. In the middle, the AI, again represented by a robot. The third person on the right will probably confuse us at first. Is it a human or a robot? The head is a human and the body is a robot. The image is intended to illustrate two challenges we face as scientists.
The first challenge concerns the interaction between AI and humans. The AI says “Ask me”, but what question should we ask, how should we formulate our questions? Different questions lead to different answers. Asking the right questions is becoming a new scientific discipline.
The second challenge is being able to differentiate between humans and AI. For example, it could happen that research proposals written by AI are evaluated by AI. This makes it clear that we need rules on what AI can and cannot be used for. Last week, the EU took a first step in this direction: after three years of negotiations, the European Parliament adopted an AI law that is intended to establish clear rules.
Karen Andes
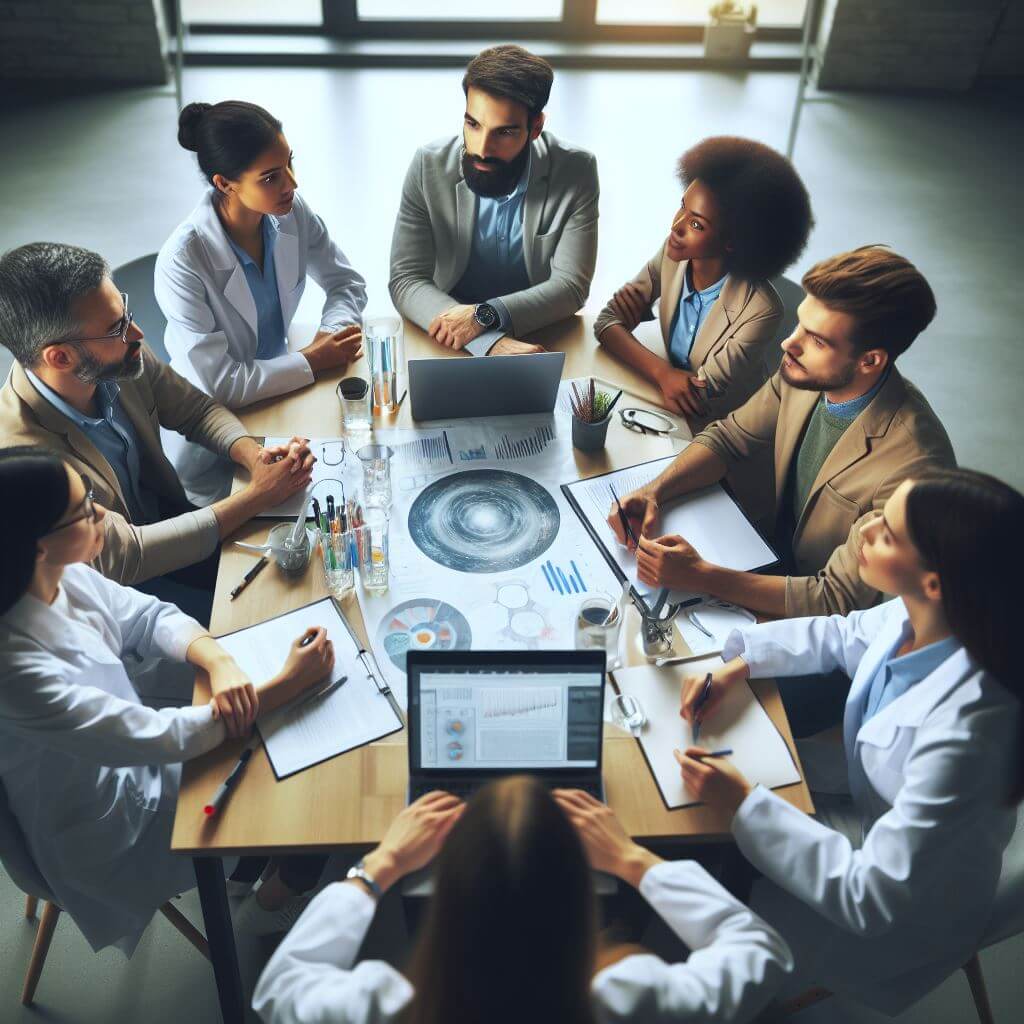
Generating this image posed a significant challenge for me, as I aimed to depict a diverse team of researchers, reflecting the nature of my work in diverse teams. However, the initial results were disappointing, with a lack of diversity in the generated images. Despite my efforts, the first iteration predominantly featured white individuals, raising concerns about representation.
In an attempt to create a scene with two peers and a mentor, I had to explicitly specify an older age for the mentor to differentiate them from the peers. Interestingly, when I requested more traditional clothing, as the peers were initially depicted in shorts on a beach, the system interpreted it as saris and clothing from various cultures worldwide. Only then did the generated images include people of color.
This experience highlights the ongoing problem of underrepresentation in research across many sectors of society. As we interact with these systems, we must exercise caution to avoid perpetuating and reiterating these disparities. It is crucial that we actively work towards ensuring diverse representation in the images we create and the research we conduct.
Stefan Rädiker
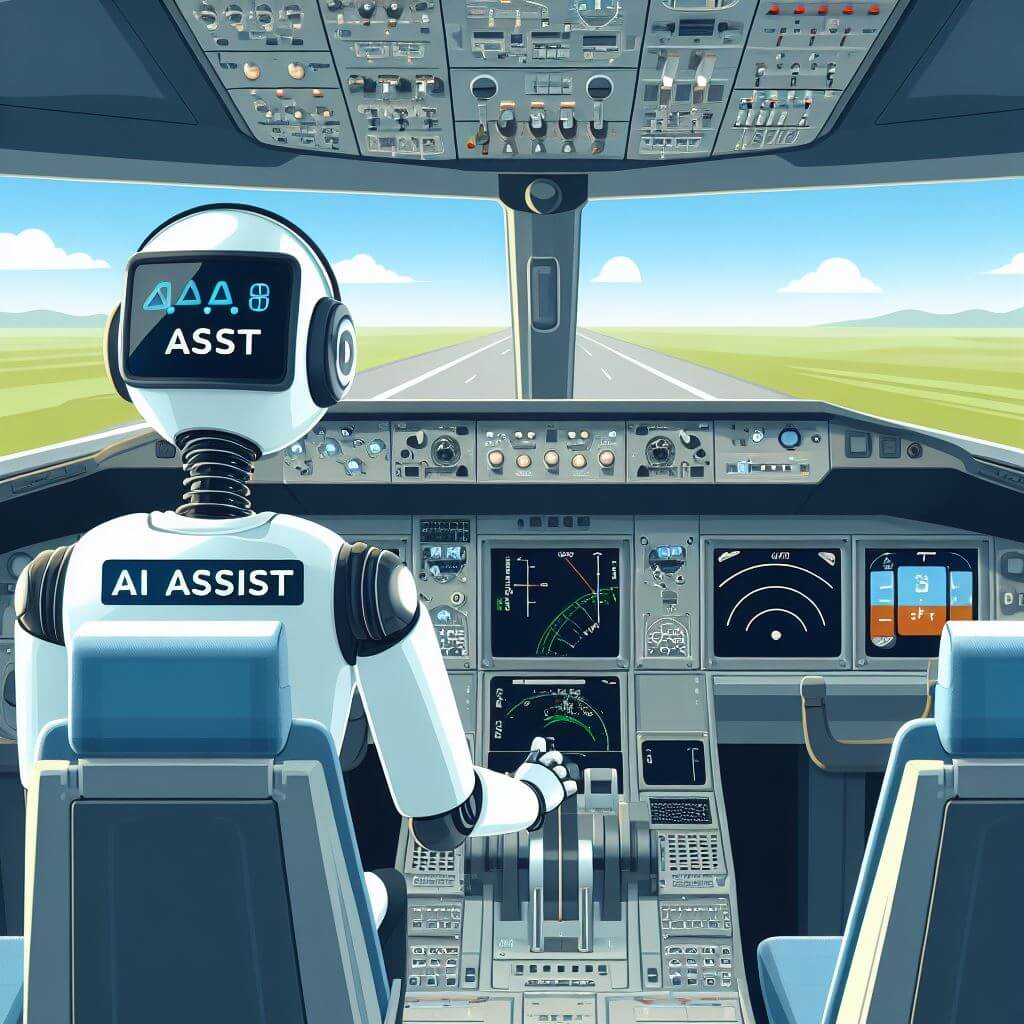
My picture raises two key questions regarding challenges:
Firstly, who is piloting the research plane? In my image, there is only a lone AI Assist robot flying the plane. Even the co-pilot seat is empty, a strong reminder of the AI’s solitary role. Interestingly, Microsoft calls several AI tools “Copilot”, but I think this is not a convincing term, and at least for qualitative research it falls too short. AI in MAXQDA is an assistant that performs tasks I’m asking it to do. In research, AI is an augmentation, an assistant or tool, aiding in specific tasks but not steering the course of research. It does not control the direction nor the destination. Actually, in qualitative research we often do not know to which destination we are heading, it’s sometimes more comparable to an adventure trip.
This leads to the second question, “What is the indispensable human factor in qualitative research?” In the film “Sully,” Tom Hanks lands an Airbus on the Hudson River in 2009. When being confronted with simulator results showing he could have landed in a nearby airport, he answers “You have taken the human factor out of the cockpit.” This underscores the irreplaceable role of human researchers in qualitative studies. Despite AI’s vast knowledge base, researchers bring invaluable real-world experience to the table, such as conducting interviews firsthand or having personal encounters with the subject matter, such as having children. Human experience is essential to qualitative research.
Discussion of Panelists
- In the “Sully” movie mentioned by Stefan Rädiker, the pilot is spoken not guilty, but it is important to notice the high reliability given to the algorithm and simulation compared to the experienced human pilot, highlighting the increasing trust in rationalization over human judgment. (Udo Kuckartz)
- When clients in research consulting confront human experts with AI-generated answers, it can lead to challenging situations. Imagine a client writing, “I have these three questions, and ChatGPT provided these answers. What’s your take on them?” If the consultant agrees with the AI, the client might question the necessity of consultant’s services. On the other hand, disagreeing with the AI could cause irritation, forcing the consultant to argue against the machine. Regardless of this challenge, the adage “two heads are better than one” still holds true. (Andreas Müller)
- AI as a second voice can also be helpful in situations where human biases and stereotypes may lead to discrimination. For example, in medical situations where women’s pain is often underrated by doctors, AI could provide a different, potentially unbiased opinion. This highlights the potential value of using AI in situations where the human factor may be discriminatory. (Julia Gerson)
- We need to distinguish between different kinds of AI, and we need to understand and learn what kinds of questions are appropriate for what kinds of models. For example, we have to be careful with GPT models when it comes to knowledge reproduction, especially book references. (Udo Kuckartz)
Discussion with Audience
- The argument of being biased is (much more) true for humans; they can be stuck in their own heads and can be very bad in taking in new information. (Audience member)
- We should not compare untrained AI to methodologically trained researchers. The goal should be to train AI to follow methodological procedures, reflexivity, and empathy, similar to how humans are trained, in order to make a fair comparison. (Audience member)
- There is a concern that using AI in qualitative research may disconnect researchers from the feelings and experiences they have when collecting data in the field. This emotional connection and researcher intuition is valuable. (Audience member)
- Each researcher is unique, shaped by their own background, experiences, and biases. AI can only assist mechanically based on the researcher’s reasoning and input. The researcher’s interpretive approach and theoretical sensitivity is still key. (Audience member)
- Data security and privacy is a major concern when uploading data to AI servers for analysis. Researchers have a responsibility to protect the safety and confidentiality of their research participants. They need to develop new technical competencies to understand how AI handles data in order to ensure security and compliance. Changes in OpenAI’s policies have already addressed several data protection concerns. (Audience member & Stefan Rädiker & Udo Kuckartz)
- There is a risk of neglecting or underestimating human natural intelligence by relying too heavily on AI-generated summaries and analyses. However, others argue AI is demonstrating capabilities that surpass individual researchers. (Audience member, Udo Kuckartz)
- Researchers’ intersectional identities shape their unique perspectives, which is especially valuable when studying populations different from themselves. AI may not be able to replicate the insights provided by diverse research teams. (Karen Andes)
- While it’s important to consider long-term implications, the current focus should be on concrete examples of how to appropriately use AI to empower and improve qualitative research within specific methodologies. Transparency about what is human vs AI-generated is crucial. (Audience member)
- AI in research can be seen as an “exoskeleton” – a tool that augments researchers’ capabilities while still leaving them in control, like a pilot. More relatable, down-to-earth examples are needed to help qualitative researchers understand the opportunities and considerations for correctly using AI. (Audience member)
3) Implementation of AI Assist in MAXQDA
– How do we make sense of the analytical output produced by AI Assist? or
– How does the workflow change when analyzing data? – now and in the future
Udo Kuckartz
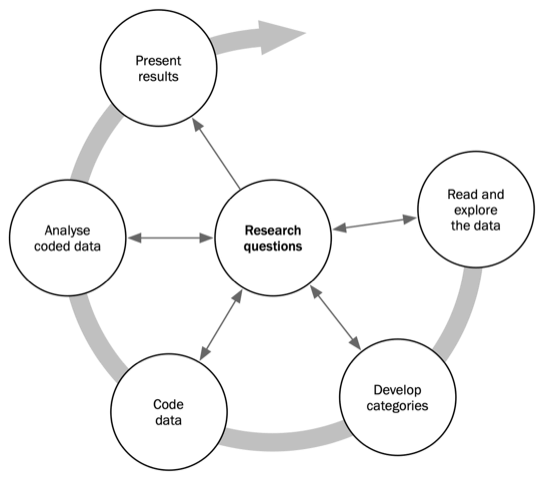
I will focus on the second part of the question, namely how the research process will change. The image comes from the book “Qualitative content analysis” (published by Sage, 2023) that Stefan Rädiker and I wrote. It shows the different phases of the qualitative content analysis method: from the initial reading and exploration of the data to the development of categories and the coding of the data to the analysis and presentation of the results.
It is the method that determines the analysis process and this remains basically the same even in times of AI, but we have to find out for each phase how AI can be used sensibly and responsibly. We have to ask for instance: “How can AI help us in creating categories?” or “What role can AI play in coding the data?”. We will see that AI can do some things very well and others less well. Clarifying these questions will lead to methodological innovations, including implementation in MAXQDA.
What applies to qualitative content analysis also applies to other established methods such as grounded theory, discourse analysis, etc. In grounded theory, for example, we can ask how AI can assist with initial coding or theoretical sampling.
Stefan Rädiker
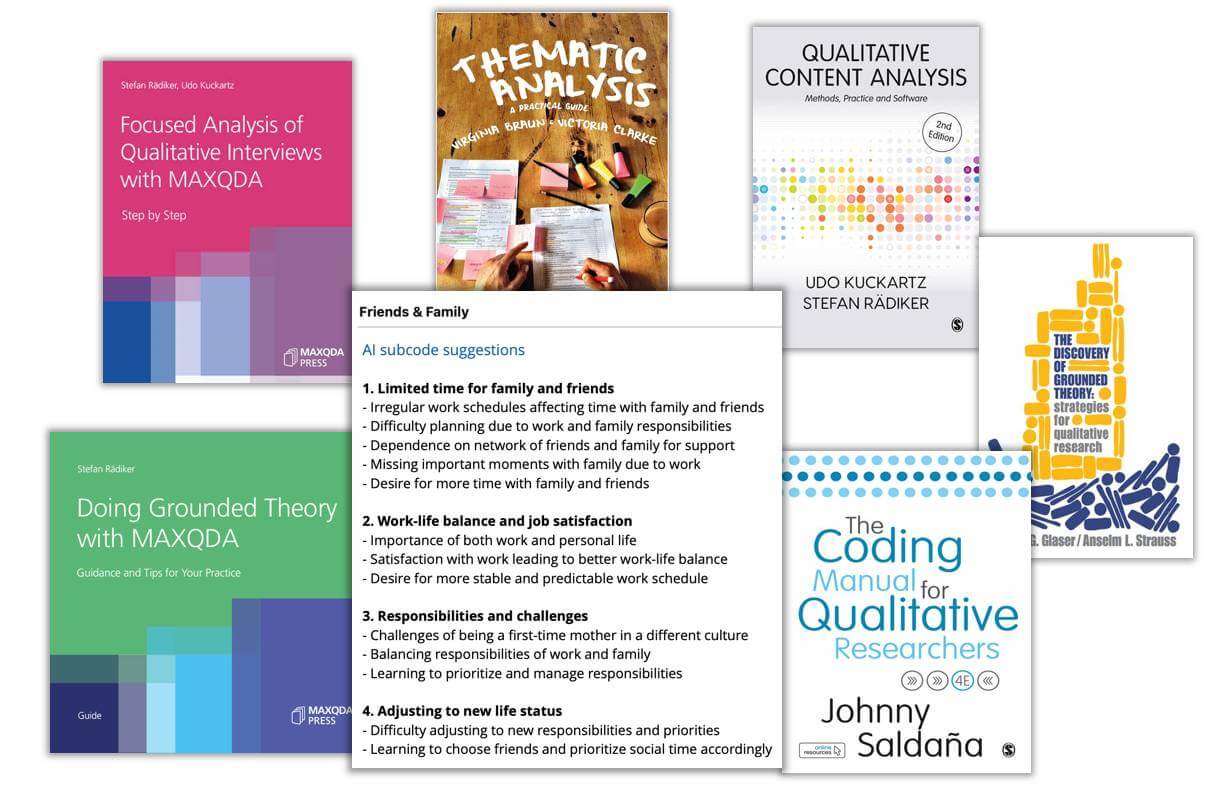
Interestingly, as Udo, I prepared an image without using AI and also focusing on research methods. It shows subcode suggestions generated by AI Assist and they are surrounded by textbooks on methods.
I was teaching a class of undergraduates who were learning to analyze interviews. We decided to allow them to use AI Assist, for example, to create subcodes and summarize the data. But we had to make sure they understood what was going on, so we asked them to write down how their results differed from the AI Assist results. We wanted to make sure that they could do this on their own, without AI Assist, and that they could reflect on the AI Assist results.
To put it simply: We need to learn and know research methods – represented by the books in my image. In fact, with all the possibilities of AI at our fingertips, I think they are more important than ever. This means reading about and practicing analysis methods to understand, evaluate and assess, improve, correct, discard, or re-request AI Assist’s outputs.
Julia Gerson
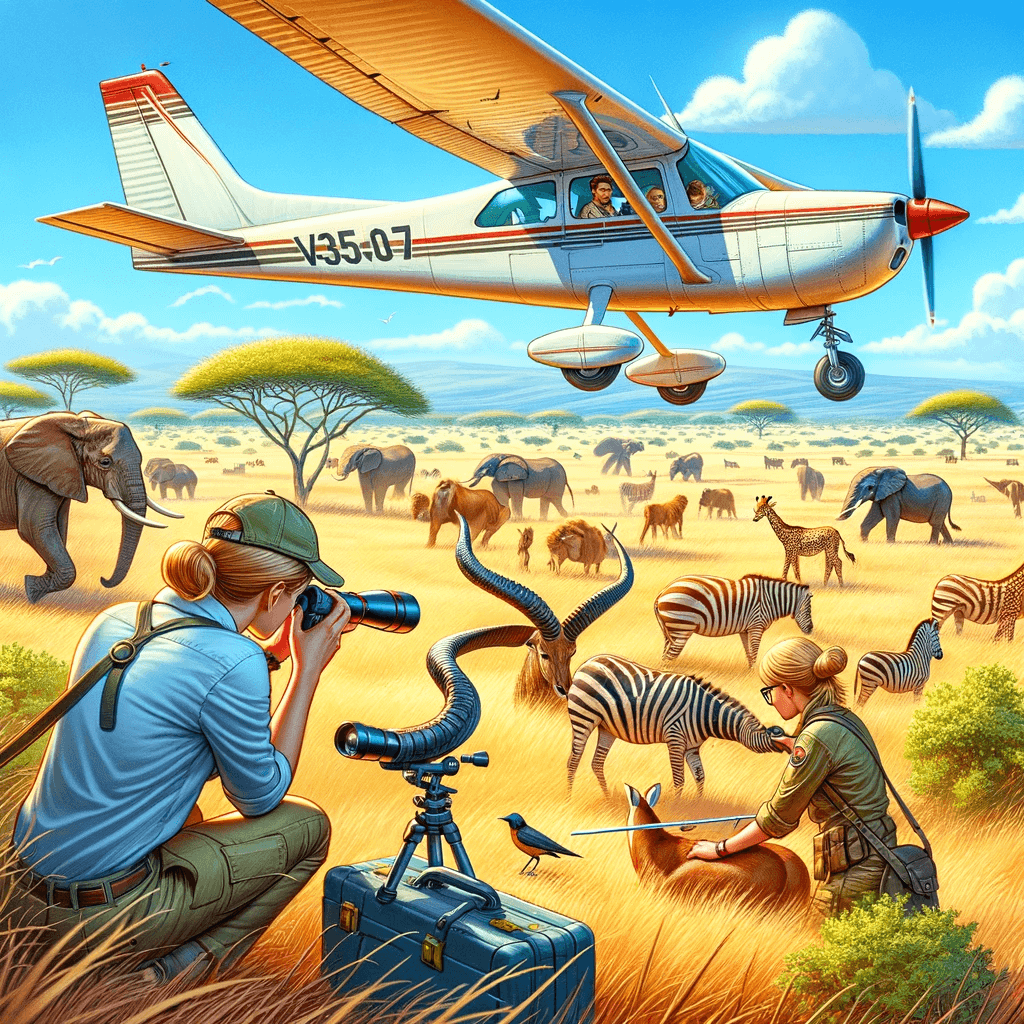
While creating this image, I wanted to focus on a potential workflow change for data collection. You can see two groups of researchers on a savanna in Africa who are apparently collecting data on animals. The two groups are using different approaches.
One group of researchers is on the ground, directly next to the animals, touching and measuring them. So, they are very close to their data, and they can feel and smell their subjects. This way they will take in a lot of details about a small number of animals.
The other group is flying over the savanna in an airplane, viewing the animals from high above. In my example, the plane symbolizes AI, which enables the user to view a much larger number of animals at once. These researchers can detect information the user on the ground cannot see, for example, a pattern in the movement of the animals, areas that are dry, and areas that are flooded.
The researchers on the plane do not have the same direct interaction with their subjects as the researchers on the ground. Still, both types of data hold value – I wouldn’t pit them against each other but rather ask how can they be combined? Different questions require different data, so researchers can decide when to use which tool and for which research questions which approach – or combination of approaches – is better suited.
While creating this image I hesitated if the image should include humans on the plane. If you put humans on a plane to fly across the land, the amount of detail they are able to process with their eyes and brains is limited and will decrease the higher up the plane goes. With AI it is different. AI can fly high across the land, collect huge amounts of data, and still notice an incredible amount of detail. So, with the help of AI, the researchers can collect vast amounts of data – but how are we going to make the data accessible to the human mind?
Karen Andes
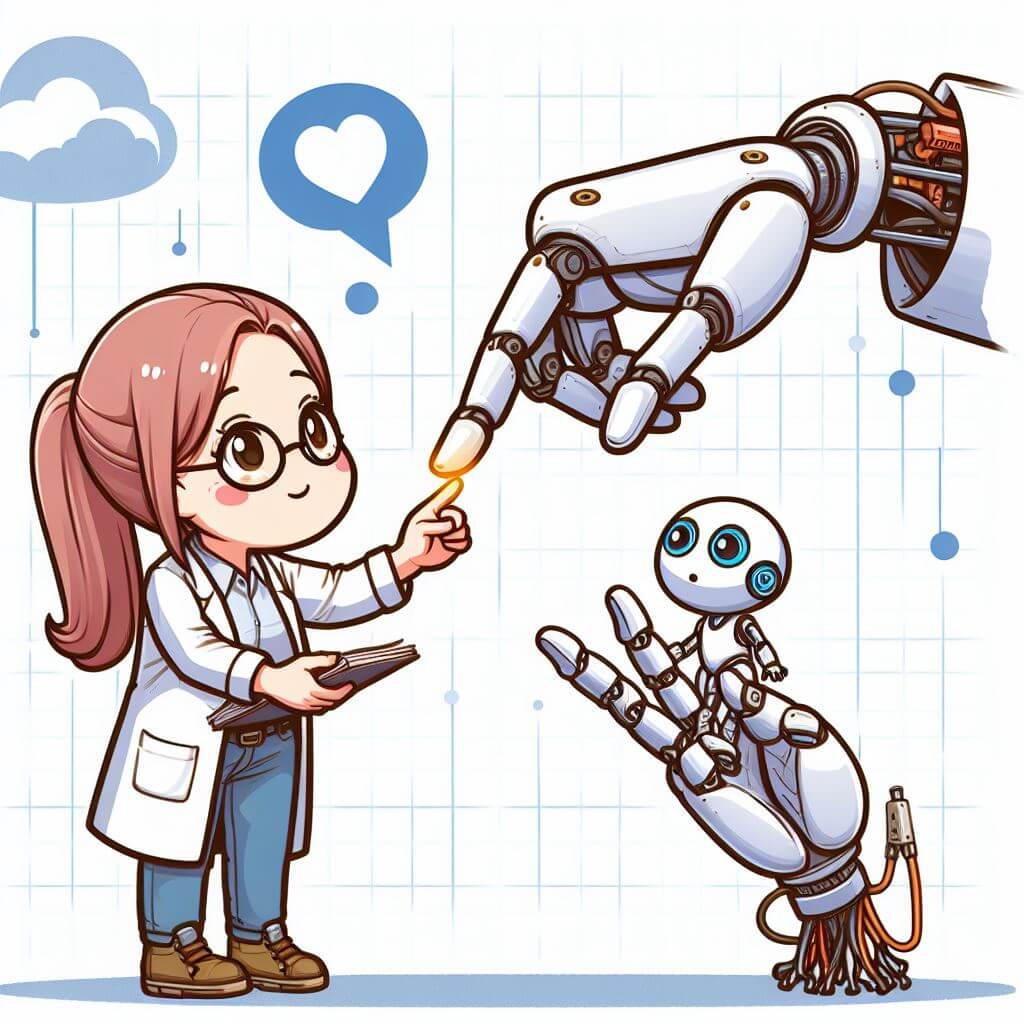
I want to emphasize that our conversation so far has been highly productive. The notion of triangulation, where AI serves as one of the pillars of our research, is particularly intriguing. It can be a powerful form of validation and support. I created an image to illustrate this concept, inspired by my own reflections on whether AI should be treated as a peer or a mentor in our projects. As AI’s capabilities in methodology continue to expand, we may increasingly view it as a mentoring figure. Within MAXQDA, for instance, you can already engage in dialogue and seek guidance, showcasing the ongoing development in this area. However, I also believe that AI can be effectively integrated into student-centered learning processes. The previously discussed approach for teaching resonates with me – having students generate their own work and collaborate in groups to review each other’s contributions. Incorporating AI as a resource in this context would not be a significant departure from my existing teaching methods, but rather an enriching addition.
Discussion with Audience
- One question is whether we expect AI in research to replicate our exact methods or if we see it as a form of triangulation – getting an opinion from someone without the same feelings and biases. This ties into the broader question of what role we want AI to play in qualitative research. It is possible to guide the AI and say, for example, “Act as a researcher” or “Act as a mentor.” (Andreas Müller & Udo Kuckartz)
- We need more time and hands-on experience to figure out how to best work with AI assistants and guide students in using them meaningfully. Students may become lazy and not engage in critical thinking about their data and methodology. (Audience member)
- Do AI and robotics free humans from boring, physical labor so we can focus on thinking? Even though we are capable of complex thought, we can also be lazy about it, especially students learning research who may not be excited about it. (Audience member)
- Another question is whether technologies like AI Assist in MAXQDA have actually made researchers more efficient and allowed them to use their time better – compared to the pre-software era in qualitative research? By some criteria, like sample size, closeness to the data, and transparency enabled by qualitative data analysis software (QDA software), qualitative research has become more efficient and gained much quality over the past 20 years. However, it depends on the criteria used. Ultimately, like with any technology, it’s up to the user how they utilize the time saved by AI. (Andreas Müller & Udo Kuckartz)
- The introduction of QDA software some decades ago has changed the way researchers work, making the process more efficient and allowing for more in-depth analysis. The efficiency gained from using software has led to higher quality research, as it enables researchers to easily combine text segments and explore new ideas that would have been difficult or impossible with paper-based methods. (Audience member)
- As technology advances, the standards for qualitative research may rise, similar to how household technology led to increased standards of cleanliness and intensive mothering. This could lead to a growing distinction between human intervention and burdensome work, with the latter never ending due to rising standards. (Audience member)
- In the private sector, the adoption of AI in qualitative research is likely to be driven by the potential for increased efficiency and return on investment, which may not always lead to positive outcomes. (Audience member)
- In the future, maybe in 20 years, AI may become advanced enough to handle the entire research process, from data input to answering the research question – and the idea that researchers will always remain in control of the process may be an illusion. (Audience member)
- The rapid development of AI makes it difficult to predict what qualitative research will look like in 5–10 years. MAXQDA has created an AI lab to research new technological possibilities, but they also consult with the methods community to ensure that any changes align with evolving research standards and maintain transparency. (Julia Gerson)
- While there are both opportunities and challenges, we should embrace AI with joy and optimism, recognizing that we are living in such interesting times and staying optimistic that we, as qualitative researchers, still play a role in the future. (Udo Kuckartz)
4) Concluding Remarks
The MQIC 2024 Symposium on the Opportunities and Challenges of AI in Research has provided a thought-provoking platform for discussing the rapidly evolving landscape of qualitative research in the era of artificial intelligence. The panelists and audience members have raised crucial points about the potential benefits and drawbacks of integrating AI tools like AI Assist into the research process. While AI can undoubtedly enhance efficiency, save time, and offer new perspectives on data analysis, it is essential to remain mindful of the unique value that human researchers bring to the table, such as their real-world experience, emotional connection to the subject matter, and ability to navigate complex ethical and methodological considerations.
As the AI world continues to advance at an unprecedented pace, it is evident that qualitative researchers must adapt and evolve to maintain their relevance and ensure the integrity of their work. The continuous introduction of new features, such as the ability to chat with documents, interviews, or papers within MAXQDA while we were compiling this document, further underscores the transformative potential of AI in reshaping the way we conduct qualitative research. However, it is crucial to approach these developments with a critical eye, engaging in ongoing discussions about the appropriate use of AI tools, the importance of transparency, and the need to develop new competencies to effectively leverage these technologies while upholding the core principles of qualitative inquiry.
As we navigate this uncharted territory, it is essential to foster a spirit of collaboration and open dialogue between researchers, software developers, and the broader methods community. By working together to establish guidelines, share best practices, and explore innovative approaches to integrating AI into qualitative research, we can harness the power of these tools to enhance our understanding of complex social phenomena while remaining true to the rich traditions and values that have shaped the field. Ultimately, the success of this endeavor will depend on our ability to strike a balance between embracing the opportunities presented by AI and maintaining a commitment to the human elements that lie at the heart of qualitative research.
This document was compiled by Stefan Rädiker supported by AI. May 2024
Download this blogpost as a PDF document: Download blogpost (PDF)
Citation (in APA format):
AI in Research: Opportunities and Challenges. (2024, February 29). [Symposium]. MAXQDA International Conference (MQIC), Berlin, Germany. https://www.maxqda.com/blogpost/ai-in-research-opportunities-and-challenges
When quoting from this documentation, please attribute statements to the persons named below each statement whenever feasible.