Guest post by Timothy Silberg.
This is my second fieldwork diary entry about my research conducted with MAXQDA, investigating socioeconomic drivers of parasitic weed (Striga spp.) control practices in Malawi. In my first blog post, I mentioned that I would try to learn more about MAXQDA’s MAXMaps feature to illuminate differences between gender-based knowledge of Striga, a weed that has emaciated maize yields in Malawi.
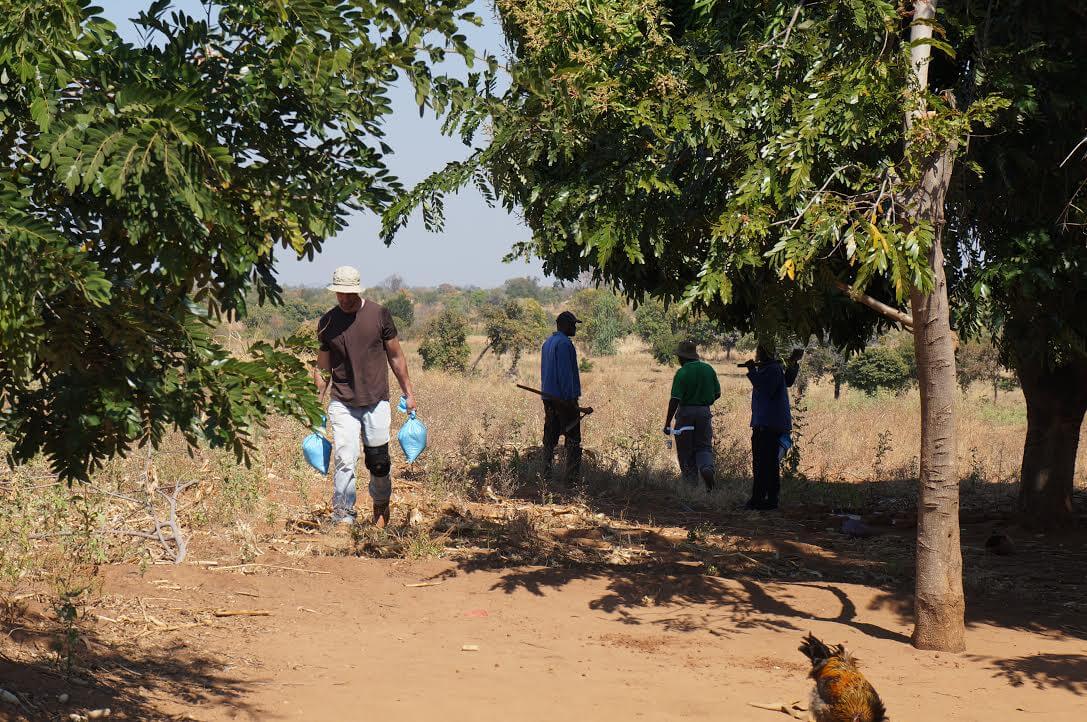
Soil Transfer Fieldwork
Gendered experiences: Farmer knowledge as it relates to practice preferences
As I became more immersed in the analysis, I was reminded of my lessons about political feminist ecology. The theory and its associated frameworks analyze how gendered experiences result from a person’s political-economic environments. In the context of Malawi, property regimes, social relations, and other components of the political-economic environment can affect farmers’ knowledge regarding Striga, and subsequently, their preference of weed control methods.
For instance, some leguminous agroforestry technologies help reduce Striga germination, but also establish property lines. Many times, unmarried mothers are less likely to adopt these technologies because trees are considered to be ‘male’ property in certain regions of Malawi. In considering these experiences, I continued my analysis with a renewed perspective of how experiences can expose different types of farmers to different knowledge; thus, affecting their preference for agricultural practices.
Code Relations Browser: Statements of Striga as they relate to gender
At this stage in my analysis, I am trying to uncover how different farmers are associated with different types of knowledge regarding Striga.
- I began by activating all “General Striga Knowledge” sub-nodes in my code system.
- I then selected the Visual Tools Tab and clicked the Code Relations Browser.
- I was given the option to choose which sub-nodes would be displayed in the rows and columns. I selected all the General Striga Knowledge sub-nodes for the row, but in the column, I checked “Choose Top Level Code” and selected “Male Respondents” and “Female Respondents”.
- Finally, I clicked the Quote Matrix icon and exported the document to Excel.
The result showed a rough picture of the gendered nuances between male and female knowledge regarding the conditions necessary for Striga germination (see Figure 1).
In this example, female farmers appeared to be more aware of the different species and their corresponding hosts. Male farmers, on the other hand, discussed more about the soil fertility requirements for Striga to germinate, as well as the weed’s extensive seed bank.
Based on this analysis, I began adding more sub-nodes in order to highlight gendered knowledge, such as “Conditions Needed – Moisture”, “Conditions Needed – Soil Fertility”, etc. With these new nodes, I conducted a more in-depth analysis of the connections between control trait preferences.
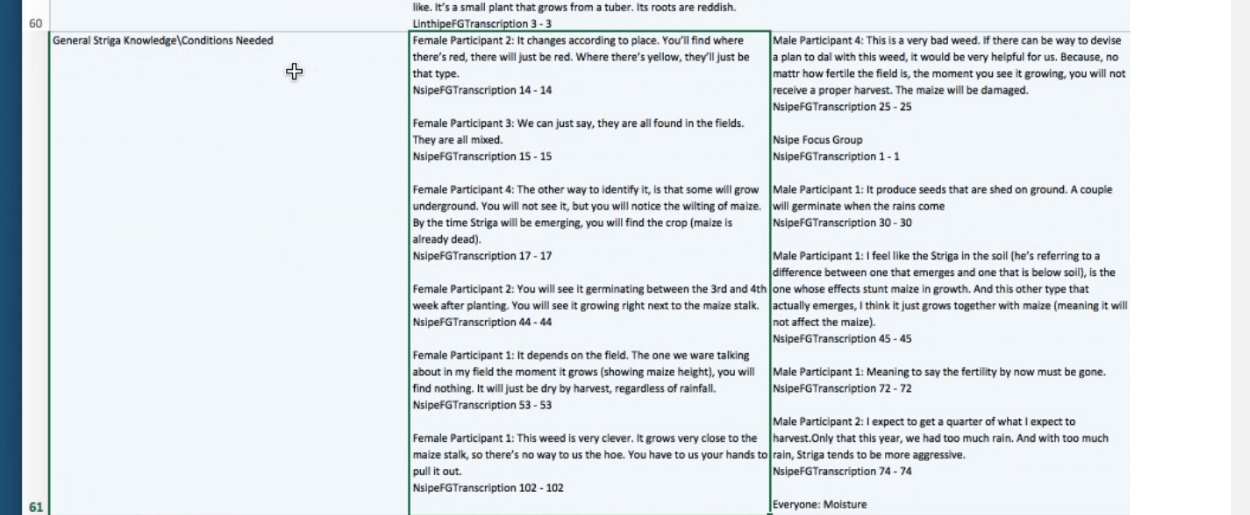
1: Assessing gendered knowledge with MAXQDA’s Reports Tool – Summaries with Coded Segments
MAXMaps: Importing co-occurring codes
I dragged these newly added sub-nodes from three focus group documents into MAXMaps in order to assess if there were any different connections between male and female farmers regarding Striga germination prerequisites (e.g., moisture), lifecycle (e.g., timing of emergence), identification (e.g., identify location prior to emergence) and effect on maize (e.g., underground parasitism).
To highlight these connections and their difference in frequencies, I right-clicked each sub-node in my concept map, selected “Import Co-occurring Codes” and checked the box “Use different line-width to reflect frequencies” (See Figure 2). In theory, the lines would show which node of Striga knowledge (e.g., Identification), control trait preference (e.g., less labor) or control method preference (e.g., manure application) had a stronger connection between male and female participants.
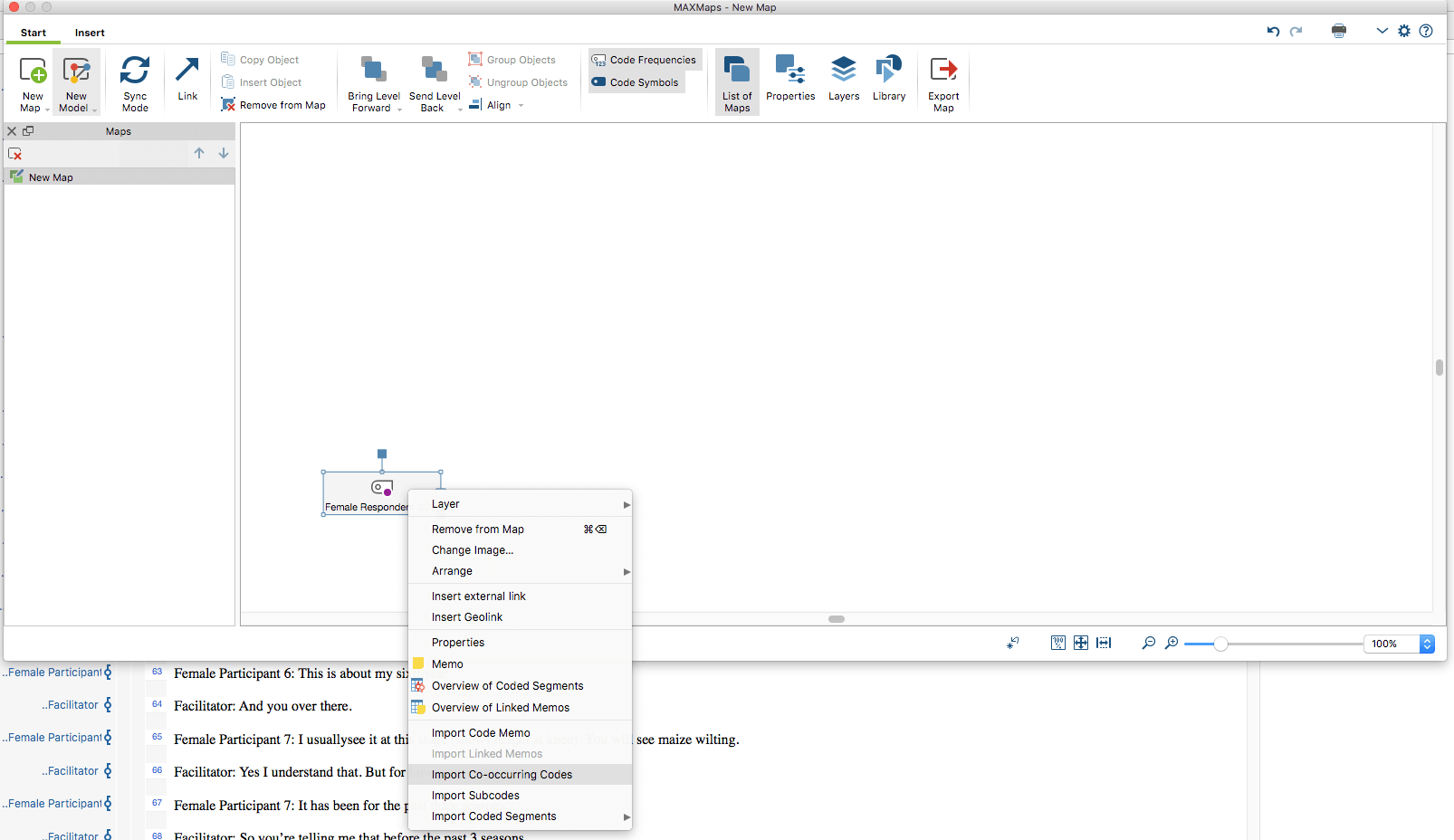
2: Import co-occurring codes into MAXMaps
MAXMaps: Line thickness to represent varying frequencies
When I created the concept map, the list was too extensive and only highlighted differences of knowledge between male and female farmers regarding broad subject areas (see Figure 3). To reassess which relationships were stronger than others, I duplicated the map, eliminated the male component and focused on female respondent connections between Striga knowledge sub-nodes.
Luckily, you can customize objects in MAXMaps. I did this by right-clicking the selected connections between nodes and sub-nodes, selecting properties and choosing a line thickness that reflected the frequency range (see Figure 4). As the line thickness properties were between 0-10 and my frequencies were between 0-10, I clicked the thickness value that reflected the frequency of each connection.
To assist possible reviewers with an understanding of the subjects regarding different knowledge nodes, I selected different shapes and colors to distinguish between groups of sub-nodes (see Figure 4). In my example, reviewers can observe that female farmers illustrate a more pronounced knowledge about the effect Striga has on maize against several other sub-nodes. In relation to this knowledge, maize yield is mentioned as one of the top three traits female farmers consider about a Striga control measure before implementing it in their fields.
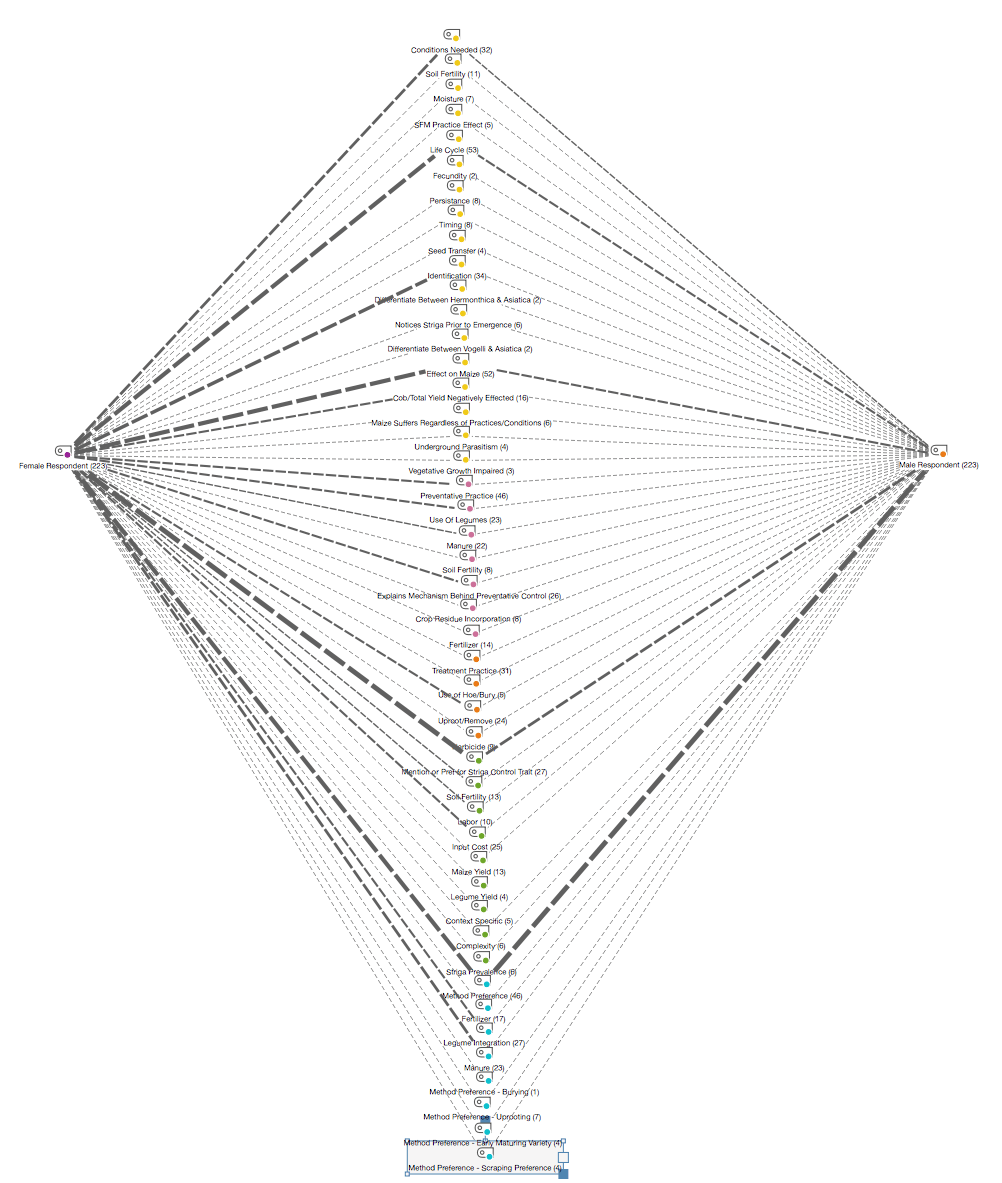
3: Gendered Striga knowledge and preferences for method traits and their control methods
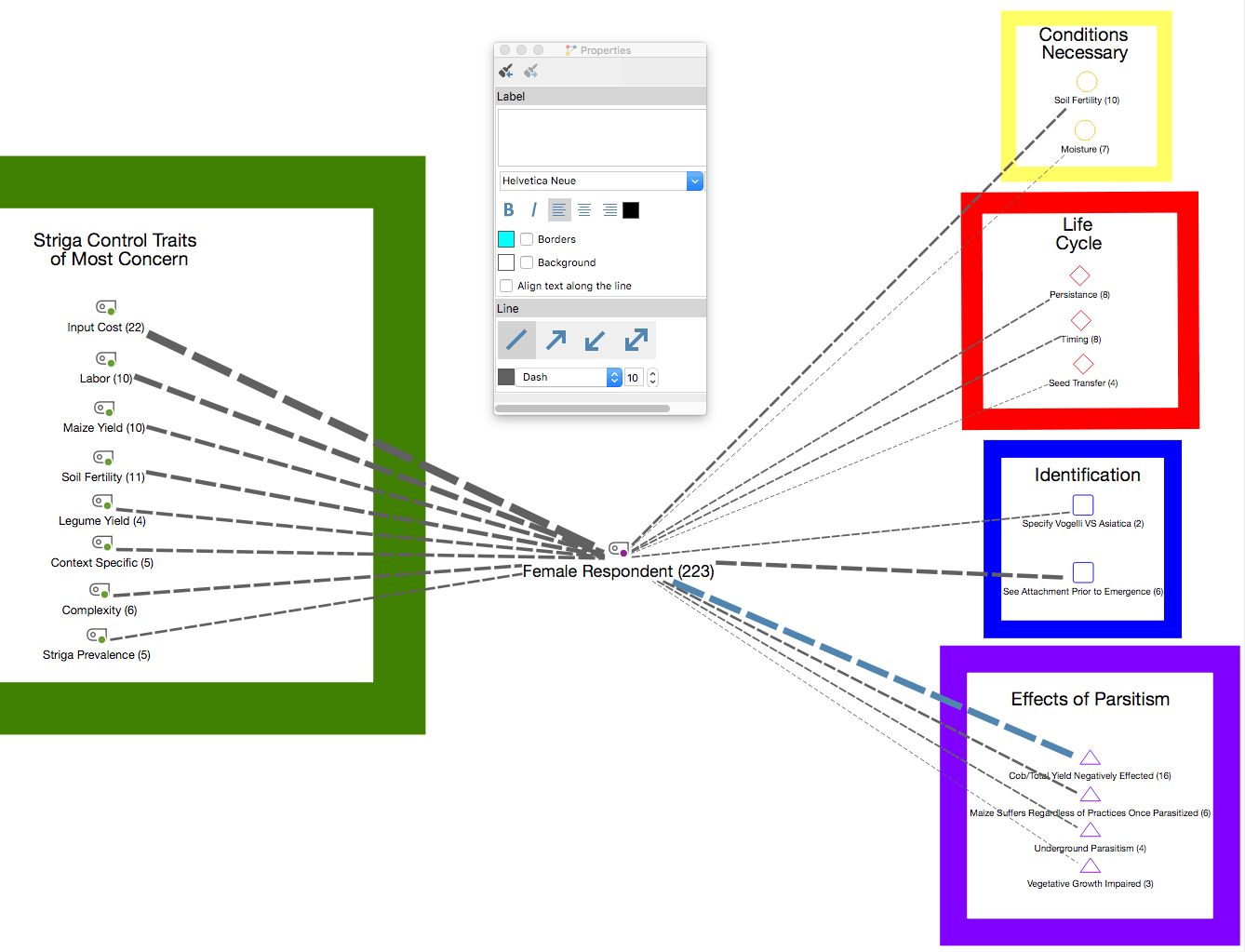
4: Striga knowledge as it relates to Striga control trait preferences
At this stage, I wanted to determine if method preferences were influenced by trait preferences for Striga control practices. I duplicated the model seen in Figure 4 and added sub-nodes that pertained to farmer-preferences for Striga control methods (see Figure 5). My MAXMaps model revealed that the control traits female farmers were concerned with appeared to be associated with method preferences (in a qualitative sense) as well. For instance, “input cost” (i.e., financial cost for employing a control method) emerged as the trait of most concern among female farmers; hence, manure, which is a relatively cost-free product, emerged as their most preferred method.
Much of my literature review confirms these findings whereby female spouses have less control over certain resources used for farm production. In some households, wives are not responsible for purchasing farm inputs in their households but their husbands are. Fertilizer, therefore, would be expected to be a Striga control practice preferred by men, which it was in this study.
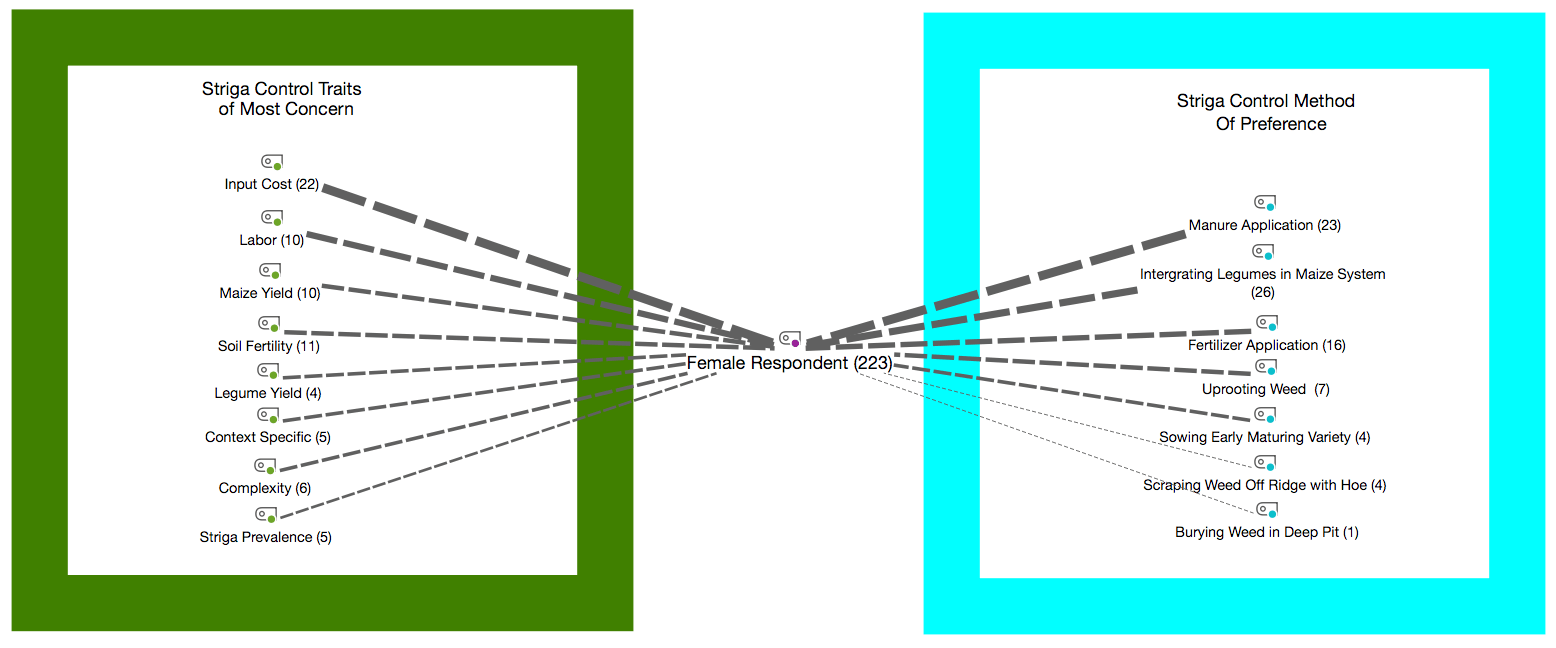
5: Gendered control trait preferences as they related to control method preferences
There are numerous Striga control practices that can be disseminated to Malawians, but without considering the knowledge and preferences of potential adopters, uptake of these practices is uncertain. The findings highlighted by this study can inform policymakers and agricultural extension agents of which Striga control traits are of most among potential adopters. The information can later be used to craft how Striga control traits are presented to different farmers to increase adoption rates.
For example, if there is a gap in the knowledge about seed bank dynamics among female farmers, extension agents can emphasize this point more in their training sessions. In addition, if labor is a concern among female farmers, perhaps extension agents can select Striga control practices that are less arduous and emphasize its labor-saving benefits.
I am excited to continue learning about MAXQDA functions I can use to highlight these gendered preferences for Striga control practices. As this analysis comes to a close, I plan to use more avenues in MAXMaps to better represent gendered knowledge as it relates to method preference. More specifically, I will try to show the exact frequencies between men and women in each sub-node. I will also attach member quotes that emphasize and articulate gendered preferences for different Striga control traits (e.g., labor, cost).
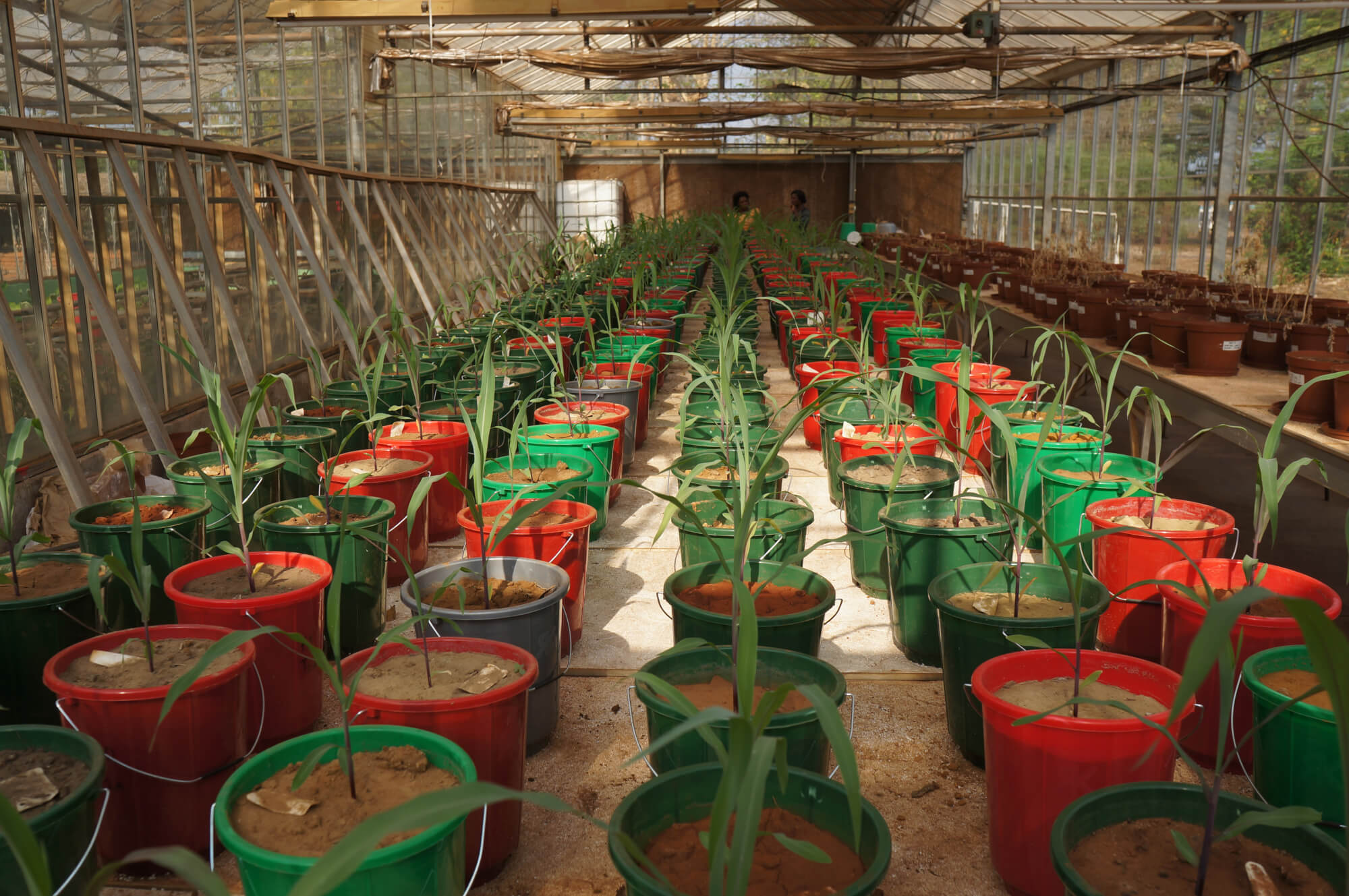
Greenhouse Trials
Editor’s Note
Timothy Silberg is a recipient of MAXQDA’s #ResearchforChange Grant and he is a PhD candidate in the Department of Community Sustainability at Michigan State University, USA. The year-long fieldwork phase of his research project titled, “Parasitic Weed Prevalence – The Case of Malawi” began in May 2017 and finished in May 2018. He has since returned to his university to conduct several analyses. This is the second of three fieldwork diary entries by Timothy Silberg. Read the first here and stay tuned for one more post from East Lansing, Michigan coming soon!