A large number of data acquired by qualitative researchers contains text with multiple speakers: a qualitative interview (interviewer/interviewee) as well as a focus group discussion, a literature analysis of a play, or a public debate. For this reason MAXQDA 12 comes with a focus group tool box that automatically recognizes different speakers during import, and moreover, implements features for the analysis of text with multiple speakers in many of its features. We’d like to give you a tour and show you how these tools can be used to explore a text with multiple speakers by looking at two recent debates of presidential candidates in the United States.
Importing a Presidential Debate – Data Preparation
Presidential debates are a perfect example for the type of material where the focus group feature offers new possibilities and can save you a lot of time and energy.
You’ll need to either transcribe a debate yourself or find an already existing transcript. A quick internet search reveals the “The American Presidency Project”. This webpage is dedicated to making more than 100,000 documents related to the Presidents of the United States available to the public. Among them are transcripts from each presidential candidate debate since 1960. We searched for the last two debate transcripts that were available (March 2016). One debate with Republican candidates in Miami, Florida (Cruz, Kasich, Rubio, Trump) and one debate with Democratic candidates in New York City (Clinton, Sanders).
As a first step, let’s create a new document for each of the debates in MAXQDA and add the text of the transcript from the webpage via copy & paste. To use the focus groups features, the transcript has to comply with a few formatting rules. The most important fact to know is that MAXQDA recognizes speakers wherever a word is followed by a colon (e.g. “Kasich:”). Luckily, our transcripts are already formatted this way, so we can transform each document into a focus group transcript by navigating to “Documents > Insert displayed text as focus group transcript”. MAXQDA 12 creates a new focus group transcript (recognizable by the orange icon), and in the process, automatically recognizes all of the speakers.
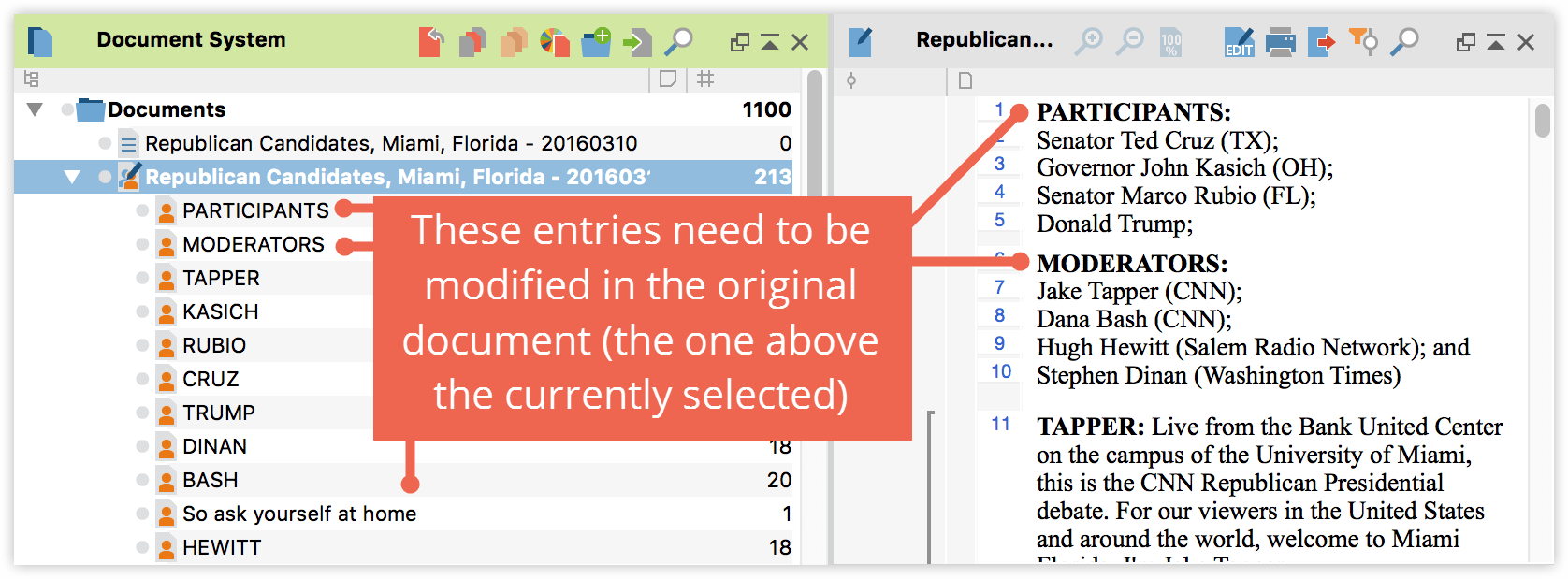
Data cleanup
In a few cases you might find that a colon was used that doesn’t signify a speaker. In our case there are two instances: 1) The preface, which lists all moderators and participants with colons, 2) A passage later on, where a colon is used to make a strong point “So ask yourself at home:”. Since MAXQDA creates a speaker icon for each speaker that is identified, you can easily search the original text document for the places where this “error” occurs and delete or replace the colons. Then delete the focus group transcript and create a new one, following the same step as described above until you only have real speakers in the speaker list.
That’s it! Time to start analyzing and getting some insights!
Exploring and visualizing
Number and length of contributions – Overview of focus group participants
Let’s start by taking a look at each participants number and length of contributions. Right click on the focus group transcript and call up the “Overview of focus group participants”.
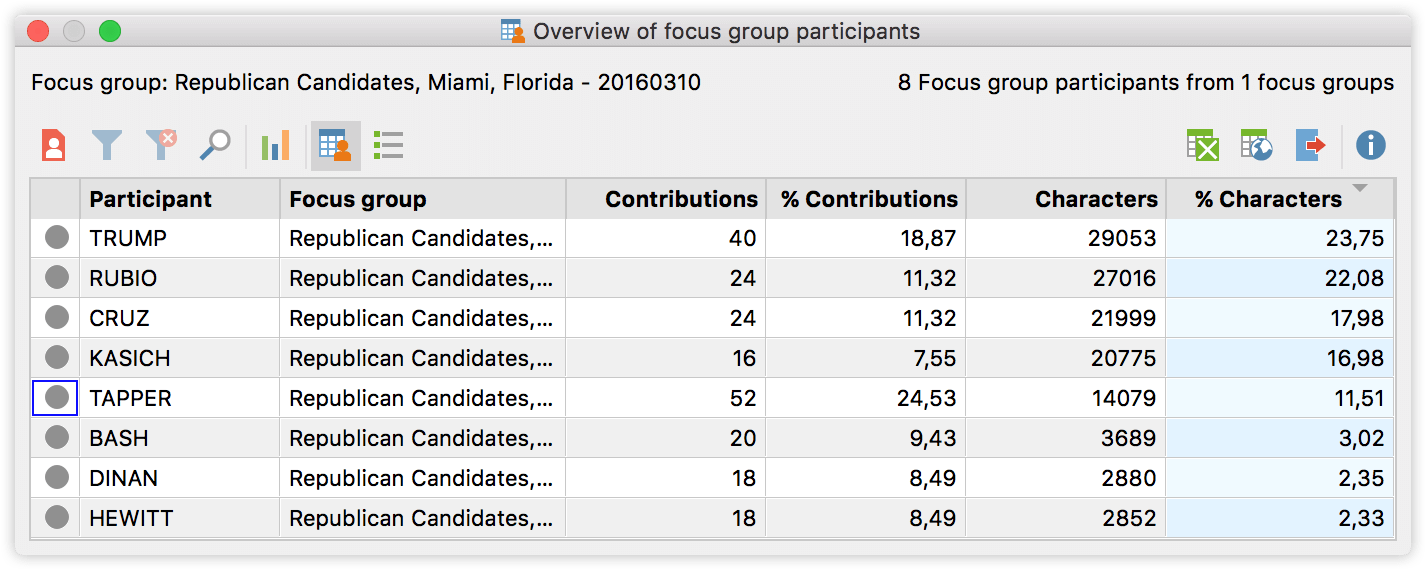
The overview tells us the number of contributions for each participant (sortable with a click on the column header). It also displays information about the number of characters these contributions contain and calculates which percentage of all the characters in the document can be attributed to each speaker. So we see for example that although Mr. Tapper (who is one of the moderators) made the most contributions, it is Mr. Trump who contributed most of the actual characters. If you want to dig deeper into these numbers you could click the statistics button, for example to find out that the mean value of contributions is 26.50.
Number and length of contributions – Document Portrait
While having statistical information about our debate almost instantly after the import is already great, sometimes it’s better to get those numbers visualized in a Document Portrait – and it’s just as simple. Go to the code system and assign different colors to each of the speakers. In our case we only colored each of the presidential candidates and left all of the moderators with the default grey color. Activate all speaker codes you want to visualize and right-click on the focus group document to select the Document Portrait.
You can either view the contributions in the order they occurred in the debate or click on “sort by frequency”. The first option gives us a pretty good impression of the course of the debate. The second option reveals that Mr. Trump and Mr. Rubio spoke more than anybody else, including the moderators and also visualizes the difference in size of all the contributions.
Speech and communication patterns – Codeline
Another way to visually explore the data is to analyze the progression of the debate using the Codeline feature. Activate all speakers you want to visualize, right click on the focus group transcript and select “Codeline”. The Codeline reads almost like a musical score. Each speaker is displayed in a single line and colored blocks are displayed wherever this speaker contributed to the discussion. It is now easy to see patterns: who engages in a discussion with whom, which role do the moderator(s) play, who makes longer, who makes shorter contributions, or who interrupts other speakers “out of turn”.
The Codeline shown above visualizes the Democratic debate. The display option is set so that the bars are colored in as soon as a contribution of this person occured in a paragraph. We can easily spot the general flow of the debate. At the start the candidates contributions go back and forth, while later on it seems that the moderator is addressing Hilary Clinton multiple times before switching to Bernie Sanders.
The Codeline from the Republican debate is set to also take the length of a contribution into account. It’s harder to see the overall pattern, but it’s easier to see which candidates answers are longer or shorter, or how long the moderators speak.
What in the name of science are they talking about?
The tools above are useful to analyze the structural characteristics and quantitative dimensions. But what about the content? An easy way to get a first impression of your data is to visualize the most frequently used words with a tag cloud, which can be created by simply right-clicking on the document and selecting the respective entry from the context menu. Let’s take a look at the tag cloud from the Democratic debate:
The most frequently used words are displayed in the largest font (e.g. Secretary, Clinton, Sanders). If you use the tag cloud for the first time, you’ll probably see a lot of words that have already been excluded from this view with a stop list (“I”, “and”, “that” etc.). You can either add these kind of words to the stop list manually, or download free stop lists from the MAXQDA webpage.
The candidates names and the word Secretary stand out in this tag cloud. But the smaller words tell us which topics seem to play an important role in the debate: America, Israel, Security, Banks, Gun, Health, and so on. What’s great about this visual display is that you can click on any of the words to get a list of all the passages in the text where this word occurs.
Here is the tag cloud from the Republican debate:
Beside the remarkable frequency with which only one of the candidate’s names stands out, it could be interesting that there are four countries among the most used words: America (46 hits – although not a country, we can assume (and later test) that this refers to the United States most of the time), Cuba (30 hits), China (20 hits) and Israel (18 hits). Let’s take a look at how often each of the candidates mentions these countries.
To do so I click on the word in the tag cloud to open the list of all passages that contain this word. With a click on “Autocode” I can then automatically code all those segments with a new code called “Autocode: [country]”. This procedure is repeated for all four words.
Now in order to visualize the occurrence of those codes for every participant, I activate each of the candidates in the list of documents, and the newly created codes. Then I open up a Code Matrix Browser from the Visual Tools menu and select “Focus Group Participants” as columns. Now we see how many times each of the candidates mentions any of the four words. The frequencies are displayed as squares, which are larger the more often the code occurs within all of the contributions of each of the selected speakers. We can now see for example, that Mr. Rubio mentioned the word “Cuba” 13 times, more than any other candidate, while Mr. Trump mentioned the word “America” two times.
Conclusion: New Features – New Insights
The focus group tools in MAXQDA 12 let you access data with multiple speakers incredibly fast. The features and steps described above are of course just a starting point. How one might use these insights and which details should be interrogated closer depends on the research projects. Also, additional types of data could benefit from these features that do not really fall into the category of a discussion group or focus group.
The automatic speaker detection could also be useful in one-on-one interview to split the interviewers comments from the interviewee. Or to differentiate between actors in a movie script. Or different user in an internet forum. We’re excited so see which kind of projects you will use this feature for. In this spirit: Happy coding!