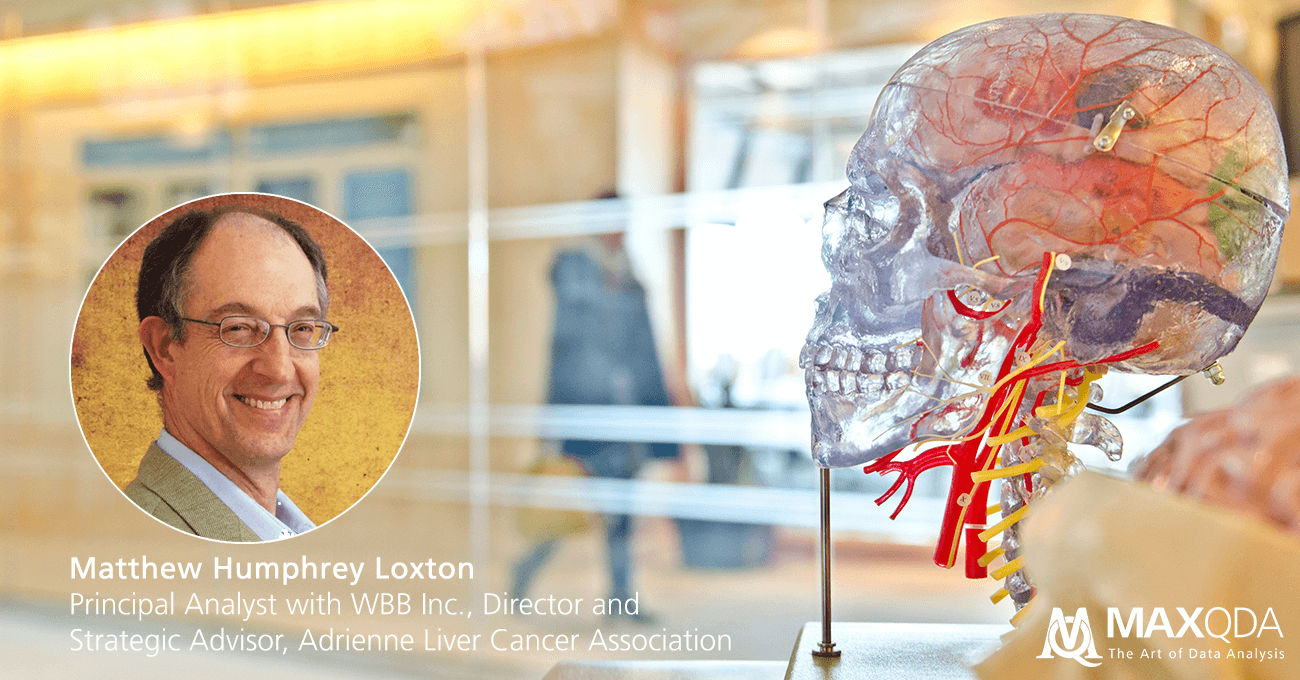
Introduction: MAXQDA and mixed methods healthcare research
Most training in research methods tells us how best to sample, process, and analyze data. However, there are times when a researcher is faced with data-collection and analysis options that are far from ideal. In practice, there may be restrictions on how data may be collected and what data are allowed to be collected. In these situations, innovative use of tools can help to produce valuable analysis even when conditions are difficult.
In this article, a team of healthcare analysts and process improvement subject matter experts from the consulting firm Whitney, Bradley, & Brown (WBB) describe an approach to a constrained data environment. In this project, the WBB team used software tools to perform both qualitative and quantitative analysis while carrying out measurement and evaluation (M&E) of the deployment of a software application across a large healthcare system. For qualitative analysis, the team used MAXQDA, and for quantitative analysis, they used R.
The preferred method of collecting user feedback on the deployment of a new Health Information Technology (IT) solution would have been to interview an appropriate number of users in 90-minute sessions and develop a survey instrument to quantify the feedback across the wider user community. However, there were data collection constraints; The team could interview only a sample of users for 30 minutes each, no survey was allowed, and the users were known to hate answering rigid questions.
The research challenge was, should the team abandon the research project because of the constraints, or conduct it in a way that would be more fluid but still produce a report that was useful, if not academically perfect?
Project Background: conducted mixed methods research with MAXQDA and R SentimentAnalysis to improve security and efficiency
The software application being assessed was implemented by a healthcare organization to improve security and efficiency of work by reducing faxing of medical documents, and enabling care coordinators, accounts clerks, and other front-line staff to exchange documents with outside organizations and healthcare providers. Since these documents contained personally identifiable information (PII), protected health information (PHI), and were often highly time-sensitive, user adoption and user sentiment were of great interest to the sponsor.
The WBB team used MAXQDA and R SentimentAnalysis to accommodate a number of constraints and still produce an analysis that was engaging and usable to the sponsor. The combination of MAXQDA to refine categories and analyze responses iteratively and R SentimentAnalysis to provide a numerical scoring of the resulting coded segments proved to be effective. This analysis gave the sponsor an insight into the risks and issues experienced during the software deployment, and opportunities for deploying and using the software in a way that further improves patient case management.
The WBB team interviewed users and asked for feedback on what was working well or not working well with the software application, the implementation process, the training they received, and the user support.
Step 1: What does the sponsor wish to know?
We held several sessions with the sponsor to document objectives and to develop a list of high-level questions. The sponsor had a small number of specific questions in mind, but for the most part, had only general thoughts and concerns. Using the text from sponsor sessions (In place of audio recording, the researchers used stenographical means to capture direct quotes from the sponsor and user sessions), we generated topics and questions and used MAXQDA to sort and classify question fragments and concerns into distinct topic categories and questions. Each question was given a code in MAXQDA in order to later associate user responses to questions. Figure 1 shows a number of questions developed from sponsor interviews and an example of how the “Notice Given” code is associated to a question and a metric.
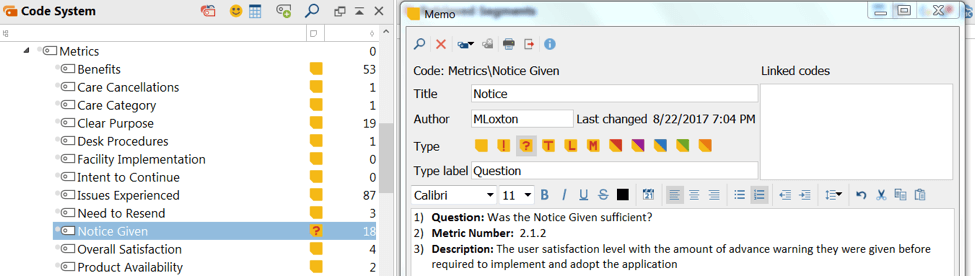
Figure 1. Question-Based Code System
Once the sponsors’ research questions were finalized, we developed a research plan.
Step 2: Research Plan
It was already known that surveys were not allowed, that interviews could take no longer than 30 minutes, and that participants would not tolerate a set of formal questions. Accordingly, the research plan was to use MAXQDA to associate text chunks from interview notes to codes already developed for each question. Figure 2 depicts the conceptual links from research questions to having a mixed-methods report that answered those questions for the sponsor. Stakeholder interviews included sessions with the sponsor, users, project members, and users. Users included case management nurses, administrative clerks, and nurse managers.
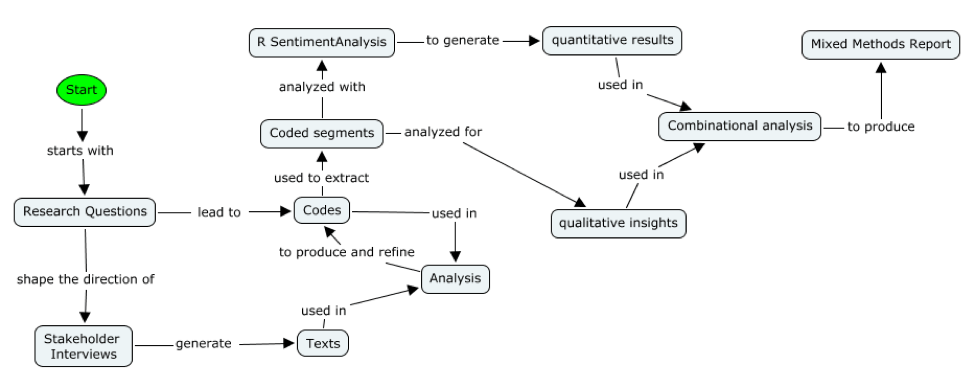
Figure 2. Research Design Flow
Step 3: Interviews
During the 30-minute interviews, the researchers remained aware of the codes as they related to the interview content and allocated codes where participant stories aligned with a question code (In other projects in which recording, MAXApp could be effectively used in the field to record and pre-code segments in this way). For example, if in response to the general prompt of “what did not work well” the participant explained that they were unaware of the software deployment until the day they were mandated to implement it, the researcher coded this as “Notice Given”. If the participant did not volunteer information for a specific question, the researcher prompted the participant with a direct question in the last ten minutes. This resulted in very few directed questions being asked and far higher comfort for the participants.
Step 4: Qualitative Analysis
The WBB team analyzed interview notes and direct quotations using MAXQDA to associate existing codes drawn from our measurement framework corpus of metrics to text segments. The measurement framework has been developed over many years of performing assessments of healthcare policy implementations, technology deployments, and workflow optimization projects.
We used MAXQDA to inductively develop new codes as they emerged from the interviews with the software users. After this initial analysis and coding, the team used the MAXQDA Weight Scores to assign a 5-point pseudo-Likert score, emulating the way it would have been scored in a survey. Using the researchers’ judgement, the scale was attributed to each coded segment. In this way, the researcher interpreted the user story content and assigned a score of 3 if the user seemed to be neutral, 1-2 if they seemed highly or moderately negative, and 4-5 if they seemed moderately or highly positive, respectively.
Figure 3 shows an example of the free coding. The code “User adoption” is highlighted, and some of the coded segments are shown with their allocated weights. A participant quote of “this is a breakthrough” is scored as a 5, since it is strongly positive.
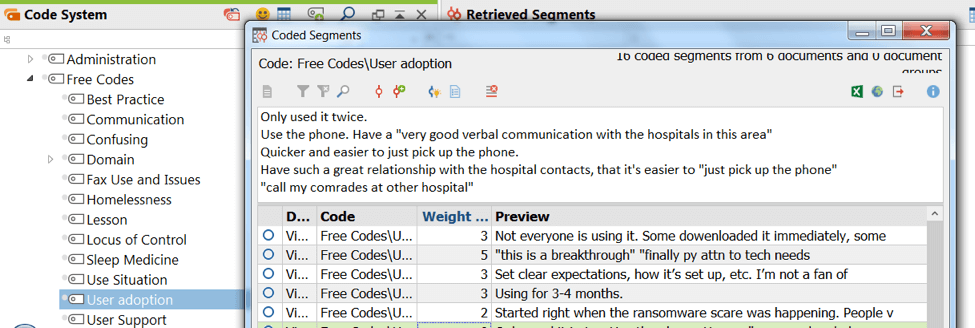
Figure 3. Free Coding and Weighting
Step 5: Sentiment Analysis in R
Once the team had classified text chunks in the partial transcriptions and interviewer notes from the semi-structured interviews, we used the R SentimentAnalysis Natural Language Processing (NLP) statistical sentiment analysis tool to quantify the degree to which the associated coded segments were positive or negative in sentiment. The R package provided a scale from -1 (strongly negative sentiment) to +1 (strongly positive sentiment) by evaluating words and phrases in the text against a dictionary of terms with given sentiment valence.
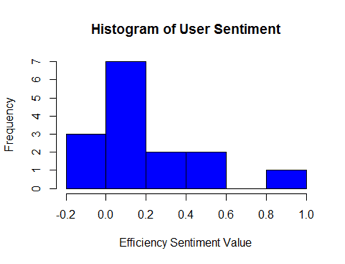
In the example (Figure 4), we associated the code “Efficiency” with coded segments in interview notes and participant quotations, and analyzed the text with the SentimentAnalysis package. In this case 15 coded segments related to efficiency, and the histogram shows that as far as the software tool efficiency was concerned, users overall felt slightly positive (mode and median above zero), with one outlier of highly positive sentiment.
We summarized the variations in sentiment scores for each appropriate code in a histogram, showing the count of coded segments for each sentiment scale.
Step 6: Combinational Analysis
The final step was to construct a report that merged the qualitative analysis developed from the coded segments with the statistical analysis of the same segments. This enabled us to describe the meaning behind the text, give an interpretation of the participant’s voice, and provide a statistical inference of the strength and direction of their sentiment. The report described what the users were thinking with regard to the deployed software, whether this was positive or negative, and how strongly they felt about it as a group.
Conclusion
Using MAXQDA to develop categories and match user stories to a sponsor’s research questions was effective in providing insight into how users perceived the deployment of a critical technology for patient management. R SentimentAnalysis provided a low-impact way to quantify user sentiment. The two together enabled a sponsor to easily identify the specific areas that needed attention, a means to prioritize them by how strongly users were likely to feel about them, and to note successes that were also strongly felt. Given data collection and analysis constraints, the stakeholder and participants were highly satisfied with the process and outcome.
About the Author
Matthew Loxton is a Principal Analyst at Whitney, Bradley, and Brown Inc. focused on healthcare improvement, serves on the board of directors of the Blue Faery Liver Cancer Association, and holds a master’s degree in KM from the University of Canberra. Matthew is the founder of the Monitoring & Evaluation, Quality Assurance, and Process Improvement (MEQAPI) organization, and regularly blogs for Physician’s Weekly. Matthew is active on social media related to healthcare improvement and hosts the weekly #MEQAPI chat. Matthew also trains others in the use of MAXQDA. You can find his contact info in his “MAXQDA professional trainer” profile.